If you’re building or growing a data science team, the first reflex is to hire new talent. Before you do so, take a few moments to ask yourself the following questions:
1. Ask Yourself The Right Questions
If your only reason for building or expanding a data science team is to “leverage your company’s data to create value and innovate,” you are off to a poor start. Instead, you should be aiming for measurable goals such as “optimizing the production line by reducing machine failures by 10%”, “reducing churn rates by x% with targeted offers,” or “analyzing and automatically clustering support ticket content to decrease time to response half”.
The following questions, and many others that will emanate from them, will be the basis to defining WHY you are building your team:
• What data will your teams be working with?
• What do you want to do with this data?
• Who is going to benefit from this data’s generated value? Directly (ie. the user or consumer of the generated value); Indirectly (ie. the organization’s bottom line)
• How much time and money can you invest in this project?
• What ROI do you expect?
Be as specific as possible when answering.
2. Technology & Tools to Achieve Your Data Team Goals
Choosing your stack and tools is crucial for your team’s future growth and performance.
That’s why you must always go back to your basic objectives rather than following the hype when deciding what technologies you’ll be needing. Sure, Spark streaming is on everyone’s lips these days. But that does not mean that your particular situation requires a system that supports streaming, or live training.
When you’re deciding what stack to build, make sure you keep short, medium, and long term needs in mind:
• Think about scalability: try anticipate how much your data is going to grow, and what new use cases you’ll want to set, or new data products you’ll want to build down the road.
• Think about accessibility & stability: remember that you’ll always have an arbitrage between accessibility and stability. For instance, if you want your data to be extremely available to perform machine learning, you’ll have to accept some loss of data and precision.
• Think about tools: scalability is important in this case as well. You want a tool that works with the different technologies you have available (or that will become available down the road). Remember that getting a team to adopt a new tool just because your stack has evolved faster than the tool itself is often a recipe for disaster. Implementing a tool that helps different technologies work together will also help different people and data profiles work together. After all, the last thing you want is to refuse an interesting hire because his or her skills don’t match your tooling environment.
3. The Hardest Game of Them All: Hiring Intelligently
Once you’ve defined objectives and what technologies will be involved, translate these into specific human components and skill sets.
1. Identify your team’s existing skill sets and what you might be missing;
2. Prioritize according to immediate needs (while keeping in mind needs that will come up down the road – remember that different profiles take more or less time to find), and build a hiring game plan of how to attract and retain future talent. Hint: from the wise words of Dataiku’s CEO, always hire people that outsmart you with skill sets you do not have;
3. Include analysts in your data science team: having analysts work with the various departments within the company to bring solid business experience to your projects is a key factor for success. Your data team shouldn’t just be data scientist superstars with extensive experience with MapReduce or Spark. For their work to be efficient, they will greatly benefit from analysts working with excel (or other such tools) who can help them build smart features with operational teams. They’re the ones that can make results accessible for business end-users throughout the company.
Furthermore, if what you’re looking for is an in-depth analysis with a lot of data preparation, you don’t need to bring in a $150k/year data scientist on board. Instead, you can hire an analyst with basic SQL or even just Excel skills, and use their business sensibility. With the right guidance and tools, this analyst can grow their tech skills all the while delivering on immediate needs.
So, before you go ahead and start interviewing dozens of unicorn data scientists, understand your goal, what you must do to get there, and look for people with a strong analytical mindsets who can build upon their existing skills.
Want To Find Out How To Take Your Analysts From Excel To Big Data? Get Your Free Guidebook.
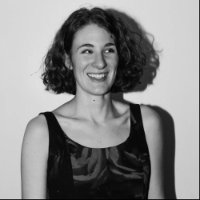
User Marketing Manager at Dataiku
Alivia Smith is User Marketing Manager at Dataiku. Alivia works with users from top American and European companies to understand how to take the pain out of data science. She is passionate about technology and is the editor for the weekly data science newsletter Banana Data News.
Speak Your Mind