In this special guest feature, Serge Haziyev, VP Technology Services Group at SoftServe, discusses how machine learning was known back in 80s as an approach to achieve Artificial Intelligence and why it took so long to achieve today’s incredible results. And what are the goals and challenges that lie ahead?. Serhiy (Serge) Haziyev is a VP Technology Services Group at SoftServe. He has more than 18 years’ experience in designing, evaluating and modernizing large scale software architectures in various technology domains including BI, Big Data, Clouds, SOA and Carrier-grade telecommunication services for both Fortune 100 and startups. He is a co-author of the architectural poker game currently used by leading institutions to teach students to architect Big Data solutions. Also, Serge is a co-author of Big Data chapter in the SEI Series book Designing Software Architectures: A Practical Approach. He frequently speaks at professional and scientific conferences across the globe (such as SEI SATURN, IEEE ICSE, WICSA and HICSS) where he conducts tutorials and provides practical inputs on emerging technologies.
It’s the last quarter of 2016, and — according to Gartner Hype Cycle for Emerging Technologies — Machine Learning is at peak of inflated expectations.
Self-driving Uber is conquering the streets of Pittsburgh, Google’s AlphaGo program defeated a human in the board game Go, Prisma app ‘repaints’ photos to look like it was composed by famous artists. These are all really impressive breakthroughs taking into account that Artificial Intelligence is quite a recent development tracing its history to 50s of 20th century.
In fact, Machine Learning was known back in 80s as an approach to achieve Artificial Intelligence. But why did it take so long to achieve today’s incredible results? And what are the goals and challenges that lie ahead?
Machine Learning in Past and Present
The 80s of 20th century were dominated by the so-called Expert Systems. While an Expert System is a computer program that emulates the decision making ability of a human expert (mostly though if–then rules), Machine Learning is based on a very different concept. The idea behind Machine Learning is that it allows computers to learn without being explicitly programmed.
Over almost 30 years, Machine Learning has been developing techniques and algorithms, gradually increasing its accuracy. For many years, performance of the majority of AI applications remained sub-human, i.e. it was worse than average human performance. But starting from 2012, the situation has started to change, and the changes are quite drastic.
Here is a little illustration of that progress based on an image recognition sample.
The bars represent an error rate of AI year over year, and the red line – the error rate of a trained human.
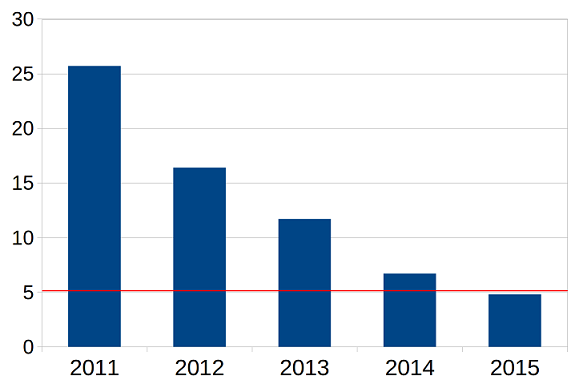
Source:https://en.wikipedia.org/wiki/Progress_in_artificial_intelligence#/media/File:Classification_of_images_progress_human.png
As you see, last year AI became par-human (i.e. it performs similarly to most humans) in image recognition.
For some tasks like driving cars AI is already super-human (i.e. it performs better than most humans), or even strong super-human (i.e. it performs better than all humans) when playing Chess or Go.
While there are several factors that made this progress possible, perhaps the major one is Deep Learning, which is a technique for implementation of Machine Learning. In just three years it enabled more than what has been done within the preceding 25 years.
Deep Learning attempts to mimic neocortex’s neurons (a part of the cerebral cortex linked to sight and hearing), which actually is a decades old idea of neural networks. The wide availability of GPUs (Graphics Processing Units, such as NVidia) made complex computations cheaper and faster, so now a hierarchy of virtual neuron layers can be deep as never before. Plus, the data massively collected over the past years including images, videos, voice and text, allows to train AI systems, so they can compete with humans on a par level or even surpass them in accuracy of recognition.
Challenges Ahead
However, even Deep Learning with unlimited computations cannot solve a general AI problem –going beyond recognizing speech or objects to perform any intellectual task, on the same level that a human being can.
The limitation lays in the nature of neural networks, which can be effectively trained for a specific function like identifying objects or recognizing words. Simply put, evolutionary we reached the level of an insect, which is a great achievement anyway. Now we need to find something that can elevate AI to the next level, enable multitasking and more abstract thinking. And there are already some promising ideas in this area like progressive neural networks and deep symbolic reinforcement learning.
While leading scientists and top companies like Google, Facebook, Amazon, Apple, Amazon, and Microsoft are heavily investing their time and budgets into the next generation AI, our modest study showed that 62% of organizations plan to adopt at least some Machine Learning for business within the next two years.
Over the next decade we will see AI being integrated seamlessly into the structure of many different organizations. The ‘sweet spot’ for Machine Learning applications varies for each organization, but considerable advantages can be gained across every sector.
Sign up for the free insideBIGDATA newsletter.
Hello I ve just seen a interesting expert panel discussion to the topics machine learning and Big Data. With guests from Microsoft, Carl data and Deloit. If you are interested in the topic you should have a look on it. 1 1/2 hours of expert knowledge 😉 Check it out: https://www.youtube.com/watch?v=Kf2oDlo9AhU&index=1&list=PLrR9xNrM9bsn6TxGeRoCiF8mqp136QKaA