Welcome insideBIGDATA AI News Briefs Bulletin Board, our timely new feature bringing you the latest industry insights and perspectives surrounding the field of AI including deep learning, large language models, generative AI, and transformers. We’re working tirelessly to dig up the most timely and curious tidbits underlying the day’s most popular technologies. We know this field is advancing rapidly and we want to bring you a regular resource to keep you informed and state-of-the-art. The news bites are constantly being added in reverse date order (most recent on top). With our bulletin board you can check back often to see what’s happening in our rapidly accelerating industry. Click HERE to check out previous “AI News Briefs” round-ups.

[12/29/2023] Mixtral-8x7B on free-tier Google Colab just dropped! Includes a novel offloading trick & mixed quantization. Notebook available HERE. Code available HERE. Paper available HERE.

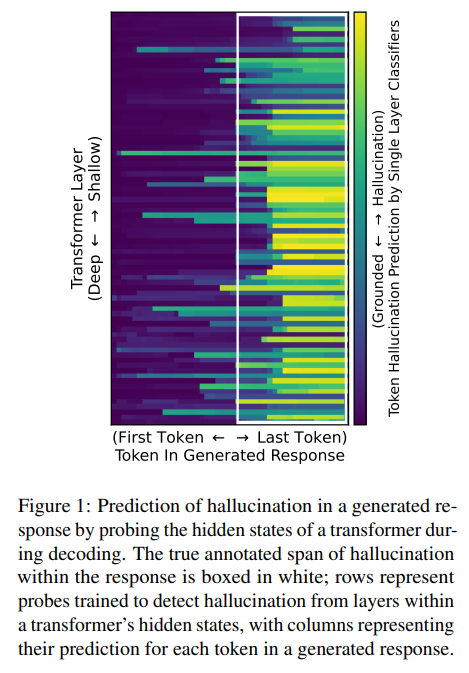
[12/29/2023] Do languages models know when they’re hallucinating? Here’s a fascinating new research paper that addresses this subject – Do Androids Know They’re Only Dreaming of Electric Sheep? The researchers from Microsoft and Columbia University designed probes trained on the internal representations of a transformer language model that are predictive of its hallucinatory behavior on in-context generation tasks. To facilitate this detection, they created a span-annotated dataset of organic and synthetic hallucinations over several tasks. They found that probes trained on the force-decoded states of synthetic hallucinations are generally ecologically invalid in organic hallucination detection. Furthermore, hidden state information about hallucination appears to be task and distribution-dependent. Intrinsic and extrinsic hallucination saliency varies across layers, hidden state types, and tasks; notably, extrinsic hallucinations tend to be more salient in a transformer’s internal representations. Outperforming multiple contemporary baselines, it’s shown that probing is a feasible and efficient alternative to language model hallucination evaluation when model states are available.

[12/29/2023] Bill Gates’ Predictions for AI in 2024 include extending its use from personal entertainment to serious professional and business applications. He sees AI as crucial in shaping the future, particularly in enhancing education, mental health, and global equity. Gates emphasizes AI’s role in redefining jobs and improving decision-making processes, and points out the Gates Foundation’s focus on using AI to address health challenges in underprivileged regions. He expresses optimism with regard to AI bridging the innovation gap between rich and poor nations, leading to a more equitable world.
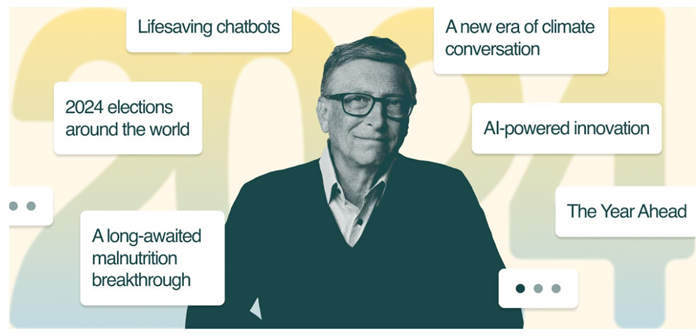

[12/29/2023] Anthropic’s 2024 Revenue Could Approach $1 Billion, According to Report

[12/28/2023] Excellent survey of 300+ research papers and summarizations of research developments in the Generative AI space. It covers computational challenges, scalability, real-world implications, and the potential for GenAI to drive progress in fields like healthcare, finance, and education.

[12/27/2023] Talk about a unicorn! OpenAI, the creator of ChatGPT, is reportedly in discussions for a new funding round, potentially pushing its valuation to or above $100 billion, as per reporting by Bloomberg.

[12/27/2023] Deloitte AI Institute’s focus on ethics in generative AI stresses the need for responsible use and risk management. Check out their new “Trust in the Era of Generative AI” report.

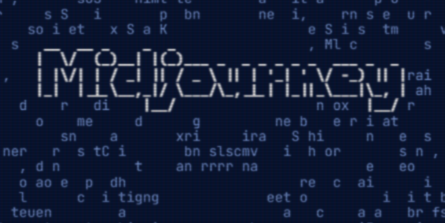
[12/27/2023] Midjourney‘s latest v6 image model is producing images strikingly close to copyrighted scenes which means the company’s infringement/conduct has gotten more questionable. V6 was released on Dec 21, but then Midjourney added this to their Terms of Service after a lot of immediate backlash, likely sometime on Dec 22/23 when people started complaining.

[12/27/2023] The New York Times filed a lawsuit today against Microsoft and OpenAI, creator of ChatGPT, accusing the companies of copyright infringement and abusing the newspaper’s intellectual property to train large language models. The Times included numerous examples in the suit of instances where GPT-4 produced altered versions of material published by the newspaper. The publisher said in a filing in the U.S. District Court for the Southern District of New York that it seeks to hold Microsoft and OpenAI to account for the “billions of dollars in statutory and actual damages” it believes it is owed for the “unlawful copying and use of The Times’s uniquely valuable works.”
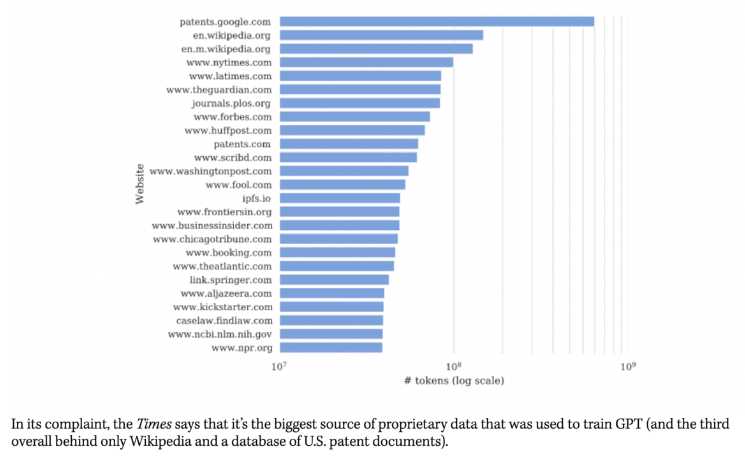

[12/27/2023] It has long been recognized that AI can achieve a higher level of performance than humans in various games – but until now, physical skill remained the ultimate human prerogative. This is no longer the case. An AI technique known as deep reinforcement learning has pushed back the limits of what can be achieved with autonomous systems and AI, achieving superhuman performance in a variety of different games such as chess and Go, video games and navigating virtual mazes. Today, artificial intelligence is beginning to push back the boundaries and gain ground on man’s prerogative: physical skill.
Researchers at ETH Zurich have created an AI robot named CyberRunner whose task is to learn how to play the popular and widely accessible labyrinth marble game. The labyrinth is a game of physical skill whose goal is to steer a marble from a given start point to the end point. In doing so, the player must prevent the ball from falling into any of the holes that are present on the labyrinth board.
CyberRunner applies recent advances in model-based reinforcement learning to the physical world and exploits its ability to make informed decisions about potentially successful behaviors by planning real-world decisions and actions into the future. The research paper can be accessed HERE.

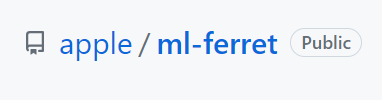
[12/26/2023] Don’t forget about Apple’s Ferret – a new open-source multimodal large language model developed with Cornell University, offers a breakthrough in AI research. Released quietly in October on GitHub without much initial fanfare, Ferret gained attention for its ability to use image regions as queries. It can precisely identify elements within an image, aiding complex queries. Ferret can refer and ground anything anywhere at any granularity. Ferret enables referring of an image region at any shape. Ferret often shows better precise understanding of small image regions than GPT-4V. See paper HERE. See GitHub HERE.

[12/25/2023] Microsoft announced WaveCoder: Widespread And Versatile Enhanced Instruction Tuning with Refined Data Generation. a fine-tuned Code LLM. Also introduced – CodeOcean, a dataset comprising 20,000 instruction instances across 4 universal code-related tasks which is aimed at augmenting the effectiveness of instruction tuning and improving the generalization ability of fine-tuned model. This research offers a significant contribution to the field of instruction data generation and fine-tuning models, providing new insights and tools for enhancing performance in code-related tasks.

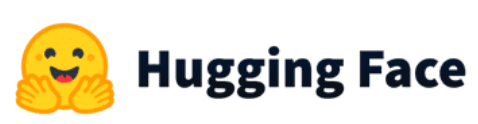
[12/23/2023] Check out an analysis of open-source large language models (LLMs) based on seven key benchmarks from the OpenLLM Leaderboard on Hugging Face, using the Eleuther AI Language Model Evaluation Harness. This harness is a unified framework designed to test generative language models across a wide range of evaluation tasks, providing a standardized approach to benchmarking. Give special focus on the following benchmarks: AI2, HellaSwag, MMLU, TruthfulQA, Winogrande, and GSM8k. These benchmarks are important for assessing LLMs in tasks related to understanding and generating human language, offering insights into their performance and capabilities.

[12/22/2023] Which countries are most interested in Generative AI? Click HERE for detailed map!

[12/22/2023] Authors Sue OpenAI – An increasing number of authors, including Pulitzer winners Kai Bird, Stacy Schiff, and Taylor Branch, joined a class-action suit claiming OpenAI and Microsoft used their content without consent to train LLM models.

[12/21/2023] 2024 alert! The coming months will focus more on multimodal models like Gemini. It’s probably a good idea to catch up on Gemini now to be better prepared for what’s coming ahead. ML and NLP research luminary Elvis Saravia has written a concise summary of Gemini and its capabilities. He promises to add more use cases and code examples soon. He’ll also perform a closer analysis of the capabilities of Gemini Ultra and Nano when made available. Read the summary HERE.

[12/21/2023] The OpenAI GPT 4.5, GPT-5 (GPT-V) rumor mill is operating with a full head of steam! What is certain is that none of this is certain. OpenAI’s Steven Heidel said “brace yourself, agi is coming.” I don’t think so!

How to Use Gemini AI by Google ✦ Tutorial for Beginners: In this video tutorial, you’ll learn how to use Gemini AI, Google’s revolutionary artificial intelligence assistant. Get ready to learn:
- What is and How to use Google Gemini AI?
- The vast array of capabilities it offers to users.
- Step-by-step instructions on how to get started with Gemini AI.
- Real-world examples of how Gemini AI can be applied to enhance your productivity and streamline your workflow.
Whether you’re a tech enthusiast, a busy professional, or simply someone who wants to explore the cutting edge of AI, this video is for you.

[12/20/2023] OpenAI is expanding its internal safety processes to fend off the threat of harmful AI. A new “safety advisory group” will sit above the technical teams and make recommendations to leadership, and the board has been granted veto power — of course, whether it will actually use it is another question entirely.

[12/19/2023] Salesforce announced it will start making available Einstein Copilot, its new all-purpose conversational AI assistant for the office. Salesforce aims to enhance its users’ customer service options and offer a new level of efficiency to sales and marketing efforts via a new interface and customizable AI models. AI-powered CRM systems like this will enable Salesforce clients to create more personalized and effective interactions without extensive work developing their own AI models. Salesforce is expecting this product will help streamline the integration of one of its key advantages: the silos of enterprise data its clients maintain on the platform. Ultimately, every so-called data aggregator like Salesforce will need to adapt to this trend to compete with hyperscalers like Microsoft and Google.

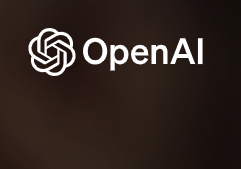
[12/19/2023] OpenAI Guide to Prompt Engineering: The guide shares 6 strategies and tactics for getting better results from LLMs like GPT-4. The methods described can sometimes be deployed in combination for greater effect. The company encourages experimentation to find the methods that work best for you. Some of the examples demonstrated in the guide currently work only with the most capable model, gpt-4
. In general, if you find that a model fails at a task and a more capable model is available, it’s often worth trying again with the more capable model.

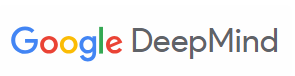
[12/17/2023] NEW from Google DeepMind! FunSearch: Making new discoveries in mathematical sciences using Large Language Model – Google DeepMind used FunSearch, a unique LLM framework, to solve a famous unsolved problem in pure mathematics – the cap set problem using a unique pairing of a pre-trained LLM with an automated evaluator for iterative solution development. FunSearch is built on a modified version of Google’s PaLM 2, termed Codey, optimized for code generation. It fills in missing solution components in a Python-sketched problem. The cap set problem involves finding the largest set of points in a high-dimensional grid where no three points align linearly. It’s a complex issue representing a broader class of problems in extremal combinatorics. The approach involved pairing a pre-trained LLM with an evaluator for program generation and refinement. FunSearch first generates a range of potential solutions. The evaluator then rigorously filters these solutions, retaining only the most accurate and viable ones. This process iteratively refines the solutions, enhancing their reliability and applicability. FunSearch outperformed existing computational methods. Access the research paper HERE.

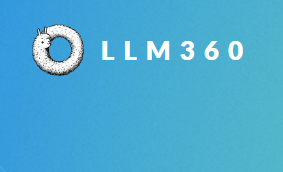
[12/17/2023] LLM360 introduced Amber-7B and Crystalcoder-7B LLMs, offering full transparency in Large Language Model (LLM) training with comprehensive open-source release including training data and code. Existing LLMs lack transparency in training processes, limiting the AI community’s ability to assess reliability, biases, and replicability. This obscurity hinders collaborative progress and thorough understanding of LLM behaviors. LLM360 tackles this by releasing two models with all training components – including 1.3T and 1.4T token datasets, training code, intermediate checkpoints, and detailed logs. Training employs AdamW optimizer, mixed-precision techniques, and thorough data mix analysis for nuanced pre-training. Amber-7B and CrystalCoder-7B demonstrate robust performance on benchmarks like ARC and MMLU. Specifics include training on diverse datasets (e.g., RefinedWeb, StarCoder), achieving 582.4k tokens per second throughput, and detailed analysis of model behaviors like memorization across training stages. Download the research paper: “LLM360: Towards Fully Transparent Open-Source LLMs.” LLM 360, a collaboration between Petuum, MBZUAI and Cerebras, is dedicated to advancing the field of AI by providing comprehensive access to large language models.

[12/17/2023] COOL PAPER ALERT! Weight subcloning: direct initialization of transformers using larger pretrained ones
Paper page: https://huggingface.co/papers/2312.09299
Abstract: Training large transformer models from scratch for a target task requires lots of data and is computationally demanding. The usual practice of transfer learning overcomes this challenge by initializing the model with weights of a pretrained model of the same size and specification to increase the convergence and training speed. However, what if no pretrained model of the required size is available? In this paper, we introduce a simple yet effective technique to transfer the knowledge of a pretrained model to smaller variants. Our approach called weight subcloning expedites the training of scaled-down transformers by initializing their weights from larger pretrained models. Weight subcloning involves an operation on the pretrained model to obtain the equivalent initialized scaled-down model. It consists of two key steps: first, we introduce neuron importance ranking to decrease the embedding dimension per layer in the pretrained model. Then, we remove blocks from the transformer model to match the number of layers in the scaled-down network. The result is a network ready to undergo training, which gains significant improvements in training speed compared to random initialization. For instance, we achieve 4x faster training for vision transformers in image classification and language models designed for next token prediction.

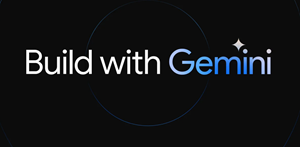
[12/16/2023] Google announced Gemini Pro developer access – its advanced artificial intelligence program, Gemini, which was unveiled last week, will now be available in a preview version for users of its AI Studio programming tool and Vertex AI, a fully managed programming tool for enterprises running on Google Cloud. Gemini will also be integrated into Duet AI, Google’s AI-enhanced coding tool, in the coming weeks. The announcement highlighted Gemini’s training on Google’s custom AI chip, the Tensor Processing Unit (TPU), and the release of TPU v5p, offering four times the performance of the existing v4 chips.

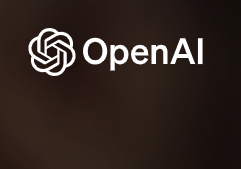
[12/16/2023] OpenAI Partnership with Axel Springer to deepen beneficial use of AI in journalism. The detail allows ChatGPT to summarize its current articles, including gated content. The deal is not exclusive and spans several years, enabling ChatGPT users worldwide to access summaries of global news from Axel Springer’s brands, with links to full articles for transparency purposes. The alignment contrasts with other media companies like CNN, the New York Times, and Disney, who have restricted their content from AI data scrapers. This collaboration is the first of its kind and aims to explore AI-enabled journalism and its potential to enhance journalism’s quality and business model.

[12/15/2023] The Mistral’s Mixtral 8x7B Instruct large language model (LLM) is now available on the OctoAI Text Gen Solution. Users can benefit from competitive quality to GPT 3.5, the flexibility of open source software, and a 4x lower price per token than GPT 3.5. Details released by Mistral AI this week provided confirmation that Mixtral implements a sparse Mixture of Experts (MoE) architecture, and provided competitive comparisons that showed Mixtral outperforming both Llama 2 70B and GPT 3.5 in several LLM benchmarks. MoE models use conditional computing to limit the number of parameters used in generating each token, lowering the computational needs for training and inference.

[12/15/2023] Meet Natasha at www.builder.ai/natasha and see how this AI slowly starts to evolve into a contextual companion across the journey and beyond. Developed by Builder.ai®, an AI-powered composable software platform for every idea and company on the planet. The AI-powered assembly line fuses together Lego-like reusable features, using Building Blocks™ automation to reduce human effort, leveraging a verified network of experts to vastly extend development capabilities, and producing apps at an exceptionally high success rate that are multitudes cheaper and faster than traditional software development.

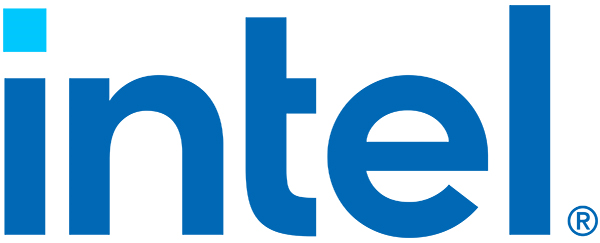
Intel unveiled new computer chips, including Gaudi3, a chip for generative AI software. Gaudi3 will launch next year and will compete with rival chips from NVIDIA and AMD that power large AI models. The most prominent AI models, like OpenAI’s ChatGPT, run on NVIDIA GPUs in the cloud. It’s one reason NVIDIA stock has been up nearly 230% year to date while Intel shares have risen 68%. And it’s why companies like AMD and, now Intel, have announced chips that they hope will attract AI companies away from NVIDIA’s dominant position in the market. While the company was shy on details, Gaudi3 will compete with NVIDIA’s H100, the main choice among companies that build huge farms of the chips to power AI applications, and AMD’s forthcoming MI300X, when it starts shipping to customers in 2024. Intel has been building Gaudi chips since 2019, when it bought a chip developer called Habana Labs.

[12/15/2023] Check out Channel 1 for “AI native news” bringing trusted news sources to the world by AI generated multilingual reporters. This is unreal!

[12/14/2023] Here are some compelling predictions for 2024 from execs at Lightning AI.
According to Lightning AI CEO Will Falcon, in 2024:
- Language models will have the same capability as they do now, for 1/10 of the parameter count
- Language models will need 1/10 of the data for the same performance
- Transformer will not be the leading architecture, especially in the lower parameter count models
- Systems that allow for multimodal AI will be predominant
- RL / DPO will enter the mainstream for open source models, alignment recipes (current moat) will be unlocked
- Boundaries between pre-training and alignment will start to blur: next token prediction on large corpora will not be the sole strategy
- Curriculum will start to be part of the (pre-)training recipe
And according to Lightning AI CTO Luca Antiga, In 2024
- RL applied to in-context learning will lead to effective agents
- Diffusion will enter the language / code space
- Companies will adopt AI meaningfully without data science / research teams
- There will be a hockey stick rise of companies including AI in their operations

[12/14/2023] OpenAI’s Superalignment team just published its first research showing a GPT-2-level model can be used to supervise GPT-4 and recover strong (GPT-3.5-level) performance. This research has unlocked a new approach to the central challenge of aligning future superhuman models while making iterative empirical progress today. Additionally, OpenAI launched a $10M Superalignment Fast Grants program, in partnership with Eric Schmidt, to support technical research towards ensuring superhuman AI systems are aligned and safe. See below my signature for more details. Please see the Generalization paper and blog posts on the research and fast grants for more details.

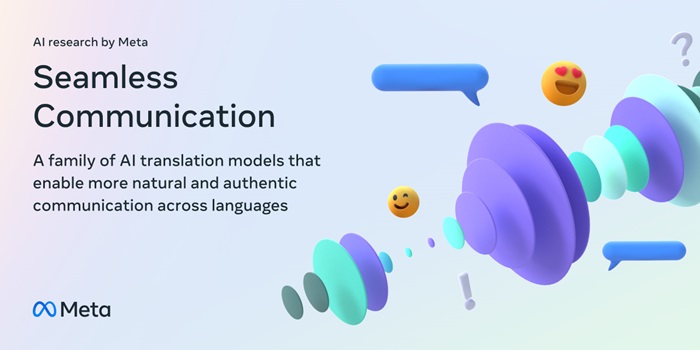
[12/13/2023] FEATURED REPO NEWS – Seamless is a family of AI models from Meta AI that enable more natural and authentic communication across languages. SeamlessM4T is a massive multilingual multimodal machine translation model supporting around 100 languages. SeamlessM4T serves as foundation for SeamlessExpressive, a model that preserves elements of prosody and voice style across languages and SeamlessStreaming, a model supporting simultaneous translation and streaming ASR for around 100 languages. SeamlessExpressive and SeamlessStreaming are combined into Seamless, a unified model featuring multilinguality, real-time and expressive translations.
SeamlessM4T v2 updates the UnitY2 framework from its predecessor and is pre-trained on 4.5M hours of unlabeled audio, and fine tuned on 114,800 hours of automatically aligned data. The architecture is optimized for lower latency, particularly in speech generation, making it more responsive and suitable for real-time applications.

[12/13/2023] At their recent first ever developer conference ModCon 2023, Modular announced Modular Accelerated Xecution (MAX): An integrated, composable suite of products that simplify your AI infrastructure and give you everything you need to deploy low-latency, high-throughput generative and traditional inference pipelines into production. MAX will be available in a free, non-commercial Developer Edition and a paid, commercial Enterprise Edition in early 2024. Check out the ModCon keynote below:

[12/13/2023] Microsoft launched Phi-2, an a 2.7 billion-parameter AI model that matches or outperforms models up to 25x larger.
“The release of Microsoft’s Phi-2 is a significant milestone,” said Victor Botev, CTO and co-founder at Iris.ai. “Microsoft has managed to challenge traditional scaling laws with a smaller-scale model that focuses on ‘textbook-quality’ data. It’s a testament to the fact that there’s more to AI than just increasing the size of the model.
Microsoft has cited “training data curation” as key to Phi-2 performing on par with models 25x larger. While it’s unclear what data and how the model was trained on it, there are a range of innovations that can allow models to do more with less. If the data itself is well structured and promotes reasoning, there is less scope for any model to hallucinate. Coding language can also be used as the training data, as it is more reason-based than text.
We must use domain-specific, structured knowledge to make sure language models ingest, process, and reproduce information on a factual basis. Taking this further, knowledge graphs can assess and demonstrate the steps a language model takes to arrive at its outputs, essentially generating a possible chain of thoughts. The less room for interpretation in this training means models are more likely to be guided to factually accurate answers. They will also require fewer parameters to generate better-reasoned responses.
AI will be transformational for businesses and society, but first it has to be cost-effective. Ever-increasing parameter counts are not financially feasible and have huge implications for energy efficiency. Smaller models with high performance like Phi-2 represent the way forward.”

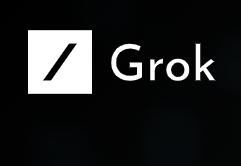
[12/12/2023] Elon Musk’s X started offering access to its new LLM (Grok) in the US. Designed to compete with other major AI models like OpenAI’s ChatGPT, Grok stands out for its integration with social media application X, allowing it real-time access to information from the platform. This feature gives Grok an edge over other AI models that generally rely on older internet data. Grok is available for X’s US Premium Plus subscribers.

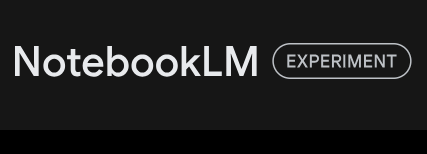
[12/12/2023] Google’s AI-powered writing assistant, NotebookLM, is transitioning from an experimental phase to an official service with significant upgrades. Initially introduced as “Project Tailwind” at Google I/O 2023, a new kind of notebook designed to help people learn faster, NotebookLM aims to organize notes by summarizing content and highlighting key topics and questions for better understanding. It’s Google’s endeavor to reimagine what notetaking software might look like if you designed it from scratch knowing that you would have a powerful language model at its core: hence the LM. The latest version runs on Google’s advanced AI model, Gemini Pro, which enhances the tool’s reasoning skills and document comprehension.

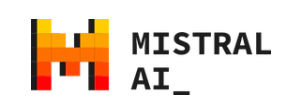
[12/12/2023] French startup Mistral AI announced it completed a Series A funding round, raising €385 million (approximately $415 million), valuing the company at around $2 billion. The company, co-founded by Google DeepMind and Meta alumni, focuses on developing foundational models with an open technology approach.
The start-up, best known for its Mistral 7B model and advocacy for regulatory exemptions for foundational models, has recently released Mixtral 8x7B and Mistral-medium models, both available through its newly launched developer platform. While Mixtral 8x7B is accessible as a free download, Mistral-medium is exclusive to the paid API platform, reflecting the company’s strategy to monetize its AI models.

[12/11/2023] Here is my favorite paper so far from NeurIPS 2023 happening this week: “Are Emergent Abilities of Large Language Models a Mirage?” It looks deep into GenAI model explainability and interpretability. The primary author, Rylan Schaeffer, did an oral poster presentation today that was quite compelling. The paper was voted one of only two “Outstanding Main Track Papers.”
Abstract:
Recent work claims that large language models display emergent abilities, abilities not present in smaller-scale models that are present in larger-scale models. What makes emergent abilities intriguing is two-fold: their sharpness, transitioning seemingly instantaneously from not present to present, and their unpredictability, appearing at seemingly unforeseeable model scales. Here, we present an alternative explanation for emergent abilities: that for a particular task and model family, when analyzing fixed model outputs, emergent abilities appear due the researcher’s choice of metric rather than due to fundamental changes in model behavior with scale. Specifically, nonlinear or discontinuous metrics produce apparent emergent abilities, whereas linear or continuous metrics produce smooth, continuous, predictable changes in model performance. We present our alternative explanation in a simple mathematical model, then test it in three complementary ways: we (1) make, test and confirm three predictions on the effect of metric choice using the InstructGPT/GPT-3 family on tasks with claimed emergent abilities, (2) make, test and confirm two predictions about metric choices in a meta-analysis of emergent abilities on BIG-Bench; and (3) show how to choose metrics to produce never-before-seen seemingly emergent abilities in multiple vision tasks across diverse deep networks. Via all three analyses, we provide evidence that alleged emergent abilities evaporate with different metrics or with better statistics, and may not be a fundamental property of scaling AI models.

[12/11/2023] Google is exploring a new application called “Project Ellman,” which proposes to use its new Gemini AI models to create personalized life stories for users by analyzing images from Google Photos and text from Google Search. The conceptual project was named after the literary critic Richard David Ellmann, and envisions a chatbot that knows everything about a person’s life, weaving a narrative from various data sources like photos and public internet information.

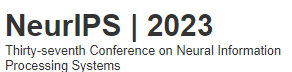
[12/10/2023] The NeurIPS (Neural Information Processing Systems) 2023 Conference starts today and runs through Dec. 16 in New Orleans. This is the premiere AI research conference of the year and has become one of my favorite events. The academic vibe is invigorating and I am looking forward to many of the sessions and research papers featured on agenda. The NeurIPS purpose is to foster the exchange of research advances in Artificial Intelligence and Machine Learning.

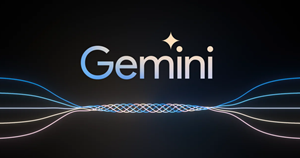
[12/8/2023] Google just unveiled Gemini 1.0, its largest and most capable AI model. Gemini was trained using the company’s custom-designed AI accelerators, Cloud TPU v4 and v5e. Built natively to be multimodal, it’s the first step in the Gemini-era of models. Gemini is optimized in three sizes – Ultra, Pro, and Nano. In benchmark tests, Gemini outperforms OpenAI’s GPT-4 in 30 of 32 tests, particularly in multimodal understanding and Python code generation.
Each model targets specific applications. Gemini Ultra, is able to perform complex tasks in data centers and enterprise applications, harnessing the full power of Google’s AI capabilities. Gemini Pro serves a wider array of AI services, integrating seamlessly with Google’s own AI service, Bard. Lastly, Gemini Nano has two versions: Nano-1 with 1.8 billion parameters and Nano-2 with 3.25 billion parameters. These models are specifically engineered for on-device operations, with a focus on optimizing performance in Android environments. For coding, Gemini uses AlphaCode 2, a code-generating system that shows the model’s proficiency in understanding and creating high-quality code in various languages.
Central to the Gemini models is an architecture built upon enhanced Transformer decoders, specifically tailored for Google’s own Tensor Processing Units (TPUs). This coupling of hardware and software enables the models to achieve efficient training and inference processes, setting them apart in terms of speed and cost-effectiveness compared to previous iterations like PaLM.
A key element of the Gemini suite is its multimodal nature – trained on a vast array of datasets including text, images, audio, and code. Gemini’s reportedly surpass OpenAI’s GPT-4 in various performance benchmarks, especially in multimodal understanding and Python code generation. The version just released, Gemini Pro, is a lighter variant of a more advanced model, Gemini Ultra, expected next year. Gemini Pro is now powering Bard, Google’s ChatGPT rival, and promises improved abilities in reasoning and understanding.
Gemini Ultra is said to be “natively multimodal,” processing a diverse range of data including text, images, audio, and videos. This capability surpasses OpenAI’s GPT-4 in vision problem domains, but the improvements are marginal in many aspects. In some benchmarks, for example, Gemini Ultra only slightly outperforms GPT-4.
A concerning aspect of Gemini is Google’s secrecy around the model’s training data. Questions about the data’s sources and creators’ rights were not answered. This is critical, as increasingly the AI industry is facing lawsuits over using copyrighted content without compensation and/or credit.
Gemini is getting a mixed reception after its big debut on Dec. 6, 2023, but users may have less confidence in the company’s multimodal technology and/or integrity after finding out that the most impressive demo of Gemini was pretty much faked. Parmy Olson at Bloomberg was the first to report the discrepancy. TechCrunch does a great job itemizing the issues with the video below.

[12/8/2023] OpenAI’s Q* model can reportedly perform math on the level of grade-school students. OpenAI hasn’t said what Q* is, but it has revealed plenty of clues. While Q* might not be the crucial breakthrough that will lead to AGI, it could be a strategic step towards an AI with general reasoning abilities.

[12/8/2023] Databricks unveiled new retrieval augmented generation (RAG) tooling to help build high-quality large language model applications. Key features include vector search to integrate unstructured data, low latency feature serving for structured data, and monitoring systems to scan model responses. By combining relevant contextual data sources, these capabilities aim to simplify productionizing accurate and reliable RAG apps across various business use cases.

[12/7/2023] Chain of Code: Reasoning with a Language Model-Augmented Code Emulator: Chain of Code (CoT), as described in a paper by researchers from Google DeepMind, Stanford and U.C. Berkeley, significantly enhances language models’ (LMs) reasoning capabilities by integrating code emulation, achieving a notable 12% improvement in performance over previous methods. Traditional LMs face challenges in accurately processing complex logic and linguistic tasks, especially when these tasks require understanding and manipulating code-like structures. CoT addresses this by allowing LMs to format tasks as pseudocode, which is then interpreted by a specialized emulator. The so-called “LMulator” effectively simulates code execution, providing a more robust reasoning framework for LMs. CoT’s effectiveness is demonstrated through its performance on the BIG-Bench Hard benchmark, where it achieves an 84% success rate, outperforming the previous Chain of Thought method by 12%. This showcases its ability to broaden the range of reasoning tasks LMs can handle.

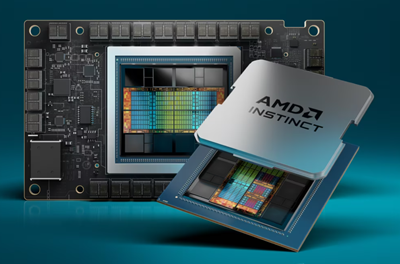
[12/7/2023] AMD (NASDAQ: AMD) announced the availability of the AMD Instinct™ MI300X accelerators – with industry leading memory bandwidth for generative AI and leadership performance for large language model (LLM) training and inferencing – as well as the AMD Instinct™ MI300A accelerated processing unit (APU) – combining the latest AMD CDNA™ 3 architecture and “Zen 4” CPUs to deliver breakthrough performance for HPC and AI workloads.
“AMD Instinct MI300 Series accelerators are designed with our most advanced technologies, delivering leadership performance, and will be in large scale cloud and enterprise deployments,” said Victor Peng, president, AMD. “By leveraging our leadership hardware, software and open ecosystem approach, cloud providers, OEMs and ODMs are bringing to market technologies that empower enterprises to adopt and deploy AI-powered solutions.”
AMD Instinct MI300X
AMD Instinct MI300X accelerators are powered by the new AMD CDNA 3 architecture. When compared to previous generation AMD Instinct MI250X accelerators, MI300X delivers nearly 40% more compute units2, 1.5x more memory capacity, 1.7x more peak theoretical memory bandwidth3 as well as support for new math formats such as FP8 and sparsity; all geared towards AI and HPC workloads.
Today’s LLMs continue to increase in size and complexity, requiring massive amounts of memory and compute. AMD Instinct MI300X accelerators feature a best-in-class 192 GB of HBM3 memory capacity as well as 5.3 TB/s peak memory bandwidth2 to deliver the performance needed for increasingly demanding AI workloads. The AMD Instinct Platform is a leadership generative AI platform built on an industry standard OCP design with eight MI300X accelerators to offer an industry leading 1.5TB of HBM3 memory capacity. The AMD Instinct Platform’s industry standard design allows OEM partners to design-in MI300X accelerators into existing AI offerings and simplify deployment and accelerate adoption of AMD Instinct accelerator-based servers.
Compared to the Nvidia H100 HGX, the AMD Instinct Platform can offer a throughput increase of up to 1.6x when running inference on LLMs like BLOOM 176B4 and is the only option on the market capable of running inference for a 70B parameter model, like Llama2, on a single MI300X accelerator; simplifying enterprise-class LLM deployments and enabling outstanding TCO.
AMD Instinct MI300A
The AMD Instinct MI300A APUs, the world’s first data center APU for HPC and AI, leverage 3D packaging and the 4th Gen AMD Infinity Architecture to deliver leadership performance on critical workloads sitting at the convergence of HPC and AI. MI300A APUs combine high-performance AMD CDNA 3 GPU cores, the latest AMD “Zen 4” x86-based CPU cores and 128GB of next-generation HBM3 memory, to deliver ~1.9x the performance-per-watt on FP32 HPC and AI workloads, compared to previous gen AMD Instinct MI250X5.
Energy efficiency is of utmost importance for the HPC and AI communities, however these workloads are extremely data- and resource-intensive. AMD Instinct MI300A APUs benefit from integrating CPU and GPU cores on a single package delivering a highly efficient platform while also providing the compute performance to accelerate training the latest AI models. AMD is setting the pace of innovation in energy efficiency with the company’s 30×25 goal, aiming to deliver a 30x energy efficiency improvement in server processors and accelerators for AI-training and HPC from 2020-20256.
The APU advantage means that AMD Instinct MI300A APUs feature unified memory and cache resources giving customers an easily programmable GPU platform, highly performant compute, fast AI training and impressive energy efficiency to power the most demanding HPC and AI workloads.

[12/7/2023] Stability released Stable LM Zephyr 3B, a compact LLM: The 3 billion parameter LLM is 60% smaller than typical 7B models, efficiently runs on edge devices, and is fine-tuned on datasets like UltraChat and MetaMathQA, excelling in Q&A tasks, benchmarked on MT Bench and AlpacaEval.

[12/6/2023] An new MIT-spinout, Liquid AI, emerged from stealth with $37.5M in seed funds at $303M post-money valuation to build a small-scale “liquid neural network” backed by Samsung Next, Bold Capital Partners, and ISAI Cap Venture.
A research paper titled “Liquid Time-constant Networks,” published at the tail end of 2020 by Hasani, Rus, Lechner, Amini and others, put liquid neural networks on the map following several years of fits and starts; liquid neural networks as a concept have been around since 2018.
Liquid neural networks consist of “neurons” governed by equations that predict each individual neuron’s behavior over time, like most other modern model architectures. The “liquid” in the term “liquid neural networks” refers to the architecture’s flexibility; inspired by the “brains” of roundworms, not only are liquid neural networks much smaller than traditional AI models, but they require far less compute power to run.

[12/1/2/2023] Deci announced the release of a new object detection model, YOLO-NAS-POSE – a derivative of YOLO-NAS, pose estimation architecture, providing superior real-time object detection capabilities and production-ready performance. Deci’s mission is to provide AI teams with tools to remove development barriers and attain efficient inference performance more quickly.
YOLO-NAS Pose offers superior accuracy-latency balance compared to YOLOv8 Pose with 38% lower latency and higher precision. YOLO-NAS-Pose performs simultaneous person detection and pose prediction in a single-pass image process along with simplified post-processing, enabling high speed and ease of deployment. With its one-line export to ONNX and NVIDIA TensorRT, conversion into production frameworks is swift and smooth. The YOLO-NAS Pose architecture is available under an open-source license. Its pre-trained weights are available for non-commercial use on SuperGradients, Deci’s PyTorch-based, open-source, computer vision training library.
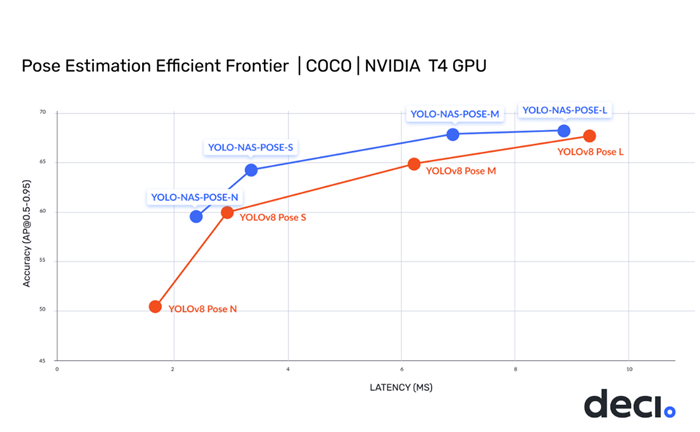
Sign up for the free insideBIGDATA newsletter.
Join us on Twitter: https://twitter.com/InsideBigData1
Join us on LinkedIn: https://www.linkedin.com/company/insidebigdata/
Join us on Facebook: https://www.facebook.com/insideBIGDATANOW
Speak Your Mind