In fields involved with knowledge production, unsupervised machine-learning algorithms are becoming the standard. These algorithms allow us to statistically analyze data sets that exceed traditional analytic capabilities. Topic modeling, for example, is gradually emerging as the strategy of choice in marketing research, social sciences, cultural analytics, and in historical, scientific, and textual scholarship.
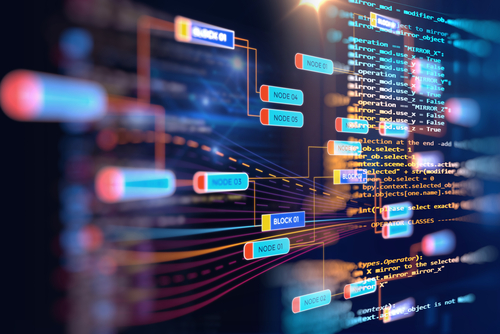
An algorithmic text-mining practice, topic modeling is used to discover recurring subjects and issues in large collections of documents. Parsing such large-scale data sets – classifying genomic sequences, mapping forms of advertisement, observing online discussions, etc. – is a matter of organization: How do you make sense of, and classify, these clusters of information?
The answer, often, is to configure them into abstract but coherent topics.
As a consequence, the software-based output of your chosen topic-modeling practice will inevitably confront you with the task of interpreting computer-generated data as texts. (Texts, in this context, being assemblages of elements of signification, or what semioticians call signs.)
Any process of interpretation of textual data relates, from this point of view, to the interplay between observable features and a specific perspective, or that which causes us see what we see during an observational process.
In developing a methodology, then, we must consider both the observed and the observer – and that starts by analyzing the problem of what we see.
The initial consideration might be that what you see in, say, the word bubbles of the DFR Browser – the graphic interface developed by Andrew Goldstone that visually renders the Mallet-processed analysis of your source texts – are not the empirical or directly observable data. They are rather estimated data resulting from a probabilistic processing of the actual words in the source material. Based on what we call posterior probability, these estimations are the influence of further conditions assigned after primary physical evidence – in our case, counting words occurrences – is gathered.
Such lists of words (topics) collectively represent just a possible picture of the object of analysis. Additionally, we need to remind ourselves that Bayesian statistics itself (on which the Latent Dirichlet Algorithm typically used in topic modeling is designed) does not work with physical probabilities but with evidential probabilities.
As a result, when it comes to topic modeling, the computer is in charge of making an initial wild “guess.” This initial guess, which is based on large-scale computations, is then followed by iterations of probabilistic hypotheses. Each hypothesis is based on occurrences and frequency – what happens and how often. Conceptually, this means that we are tasking a computer to use data to make subjective or speculative judgments.
As a collection of words, a topic radiates meaning in different ways to different people. The same is true with different settings and purposes.
To this gigantic algorithmic speculation, we then add the one connected with the human-reading practice (how we identify or label a topic as part of a specific area of meaning).
We can gradually begin to understand how the process of “topic labeling,” far from being the result of any possible automated or standardized procedure, represents the final stage of speculative layers at both the machinic and human level.
It might therefore be useful to assume that a topic is always within the realm of a “possibility of signification,” a textual status that the humanities, and literary theory in particular, have extensively addressed over the past fifty years.
As a collection of words, a topic radiates meaning in different ways to different people. The same is true with different settings and purposes. We can understand, then, how the remarkable amount of scholarship on meaning ambiguity and language polysemy typical of the humanities can come as an extremely valuable help and actual operational toolkit for contemporary data science.
As the use of topic modeling techniques is likely to become more and more widespread, the problem of data-based identification of topics will increasingly become a central one. This will require the implementation of theories of interpretation that literary studies and humanistic scholarship have refined across their centuries-long traditions of studies.
About the Author
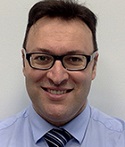
Dr. Mauro Carassai — the academic lead of California State University, Northridge’s Master of Knowledge Management program — explores the importance of humanities to the data sciences.
Speak Your Mind