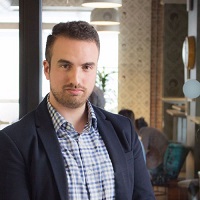
In this special guest feature, Sinan Ozdemir, Director of Data Science at Directly, points out how algorithmic bias has been one of the most talked-about issues in AI for years, yet it remains one of the most persistent challenges in the field. Sinan manages the AI and machine learning models that power the company’s intelligent automation platform. Directly has taken the messy world of making virtual agents work and reduced it to 5 simple API calls to our platform. Sinan is a former lecturer of data science at Johns Hopkins University and the author of multiple textbooks on data science and machine learning. Additionally, he is the founder of the recently acquired Kylie.ai, an enterprise-grade conversational AI platform with RPA capabilities. He holds a Master’s Degree in Pure Mathematics from Johns Hopkins University and is based in San Francisco, CA.
Ethical data processes are an essential part of creating and delivering effective training for artificial intelligence algorithms. AI model performance is closely tied to the quality of the data the model is given. When they’re built with flawed or biased data, the model doesn’t just replicate those defects — it magnifies them. Minuscule errors in training data sets become exponentially larger through programmatic computation. Subtle cultural biases are systematically amplified through automation.
Data scientists who wish to deliver an impartial product must ensure their data sets are accurate and objective, but that’s easier said than done. Algorithmic bias has been one of the most talked-about issues in AI for years, yet it remains one of the most persistent challenges in the field. Despite years of research into bias detection and mitigation strategies, it’s still easy for even the most sophisticated organizations to get into trouble for biased outcomes in their AI applications.
The Consequences of Algorithmic Bias
Last September, researchers found that the algorithm used by a large academic hospital to assign especially sick patients to an “extra care” program was systematically discriminating against black patients. The algorithm assigned patients to the program by using historical data to estimate new patients’ future healthcare costs, working on the assumption that greater costs correlate with more severe illness. However, it was later found that many black patients in the historical data set had incurred relatively low healthcare costs not because they were less sick, but because they lacked the resources to pursue adequate treatment. This resulted in a 50% reduction in black patients admitted to the extra care program, with many excluded patients having conditions that were all but identical to white patients who were admitted.
In November, entrepreneur David Heinemeier Hansson took to Twitter to complain of algorithmic gender bias in the application process for the Apple Card. Both David and his wife Jamie applied for the card, but David was given a credit limit over 20x greater than Jamie’s — despite their joint tax filings and Jamie’s higher credit score. Due to the black-box nature of Apple’s decision-making algorithm, customer service representatives were unable to explain the discrepancy. Apple’s application process is now being reviewed by federal regulators.
A month later, researchers published a study that found measurable bias in the overwhelming majority of facial-recognition systems. According to the study, facial recognition systems incorrectly identified black and Asian faces up to 100x more often than white faces, exhibited greater difficulties identifying womens’ faces as opposed to men, and falsely identified older adults up to 10x more often than middle-aged adults. While the reasons for these discrepancies is unclear, they are likely at least partially related to the data sets used to train facial recognition algorithms, which often over-represent the faces of middle-aged white males.
Bias In. Bias Out.
Ultimately, algorithmic and data bias remain an enduring challenge for data scientists for the same reasons that make systemic bias an enduring challenge for humanity. Humans are hardwired for bias. Our cognitive biases seep into every aspect of society — healthcare treatment, financial assessments, hiring practices, criminal justice — the list is virtually endless.
It’s no surprise, then, that bias would also seep into the data our society produces. This data merely describes a world with bias built into it from the start. And just as social bias begets data bias, data bias begets algorithmic bias. As AI takes over more decision-making processes,the algorithmic bias may then reinforce our implicit social biases — a vicious cycle.
There’s no one solution that will enable us to identify and remove all bias from our algorithms, and from the data sets that we use to train them. Instead, data scientists must take a proactive approach to understanding bias, evaluating it in their systems, and working to minimize its manifestation in system outputs.
How Humans and Machines Can Help Each Other
There are three key actions that data scientists should take to mitigate bias in their work:
Learn to ask the right questions. Develop a more sophisticated understanding of cognitive bias in data sets, and study approaches developed in the social sciences for identifying, interrogating, and removing biased data. In addition to a general understanding of bias, domain expertise is also crucial. For example, data scientists applying AI to healthcare should work closely with healthcare professionals to understand how bias manifests in that field.
Always test for bias. Use bias as a key metric for evaluating both raw data algorithms. All algorithmic training and testing should include a review process that gives developers a chance to aggressively test data sets and the algorithms for bias, and developers must establish appropriate criteria for measuring that bias.
Explore algorithmic solutions. Continue to develop and refine algorithms that are designed to evaluate bias in data. A great deal of progress is already being made on this front, and given time, these algorithms can help identify biases that are so deeply ingrained within us that we may have never identified them ourselves.
For now, artificial intelligence is not sophisticated enough on its own to solve the problem of bias itself. It needs training from human experts, and careful guidance from thoughtful data scientists, to deliver outcomes that are fair and equitable.
Sign up for the free insideBIGDATA newsletter.
Speak Your Mind