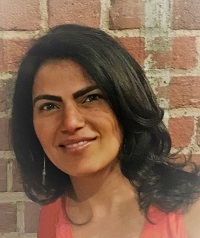
In this special guest feature, Simran Bagga, Program Director – AI and Machine Learning at Concord Technologies, take a holistic look at ways to complete a successful AI project through building the right team. Concord Technologies is a leading provider of cloud-based fax and intelligent document automation software for healthcare providers and enterprises in other regulated industries. Simran spent the last 18 years designing data science solutions to solve business problems. In her current role, she uses AI technologies to help improve efficiencies in document processing in healthcare.
Today, every organization in almost every industry is keen to leverage the power of artificial intelligence (AI) to better understand its business, clients, products, and processes. The applications of AI continue to grow. Data is everywhere, but to make it speak we need the right project goals, mindset and resources.
Being a black belt in martial arts helped me reinforce six core life skills in my personal and professional life – belief, communication, respect, honesty, self-esteem, and discipline. As I continued to train, I realized that AI project principals are no different. Believing in yourself and your team members; communicating progress, success, and any fall-backs; respecting one another’s inputs and opinions; being honest about the possibilities and timelines; enabling self-growth while maintaining integrity; and having the right discipline and attitude are all elements that contribute to a successful AI project. I bring all these considerations to my work, including how I build the team for a particular project.
The key roles
The roles on the AI team should be determined by the company’s business processes around defining and creating solutions to problems. Just like any other investment, AI should be business-led, technology-enabled. Starting with a business problem or goal is the first step to understanding the project scope. This is why an executive sponsor or decision maker of the project is a key role that helps articulate what the business is trying to accomplish and why.
Another important role on the team is that of a domain or subject matter expert (SME) who combines the in-depth understanding of the application domain, such as healthcare, with the insights from AI so that pertinent data can be leveraged for AI applications and the most impactful and relevant results can be integrated into existing systems.
Other key roles include data scientists, also known as AI engineers, who work with SMEs to build and deploy natural language processing and machine learning models. A data annotation specialist annotates and labels data in case the business has not previously stored such information. An enterprise or solutions architect may be needed to assist with technical implementation and integration if the product or service has enough interacting components to require it, or the data flows and interactions between discrete systems are complex enough to warrant it. A project owner can help ensure the success and timeliness of a project by removing any impediments, facilitating key events and shielding the team from external interruptions.
Once the team roles are determined, the question I always ask is: Can this team solve the problem effectively, while keeping the core value of the solution – the “why” – intact from inception to completion? After understanding the “why” of the AI solution for the business, the “what” and the “when” need to be fleshed out before starting the project. I work with stakeholders to understand the following questions: What problem are we trying to solve? What data do we have available? What is the timeline of the project? And most importantly, when does the solution need to be in production?
All these factors determine the skills and number of resources required for an AI project. I create requirements for the team, identify data dependencies such as annotations or data preparation needs, and define priorities. The data scientists and the solutions architect brainstorm on the “how” of the solution and discuss implementation details. They collaborate with the quality assurance engineers to identify testing scenarios so that test cases can be automated as much as possible. The detailed implementation plan along with a timeline and any associated risks are discussed and agreed upon with appropriate stakeholders. I recommend following agile methodology throughout the project and periodically review the approach, progress and results to ensure that the core value of the solution stays intact and the team delivers what’s most impactful for the business.
Throughout the project life cycles, I also keep in mind the interactive and iterative nature of AI. To enable team members to collaborate regularly and effectively, it is important to equip them with tools, technology and processes that provide value out of the box. Removing complexity while creating a flexible and scalable environment helps me lay the groundwork for success for the current and future AI applications.
A good starting point
An organization that is just beginning to harness the power of AI needs to understand how AI works and the potential business impact of the investment. Business leaders should know what’s possible given the combination of business needs, available data, and the people, processes and technology to put that data to work.
For an immediate project at hand, I suggest building the smallest team that can still be effective while giving due consideration to managing risk and unknown events. An SME is typically a resource within the organization who is familiar with a variety of data and its meaning to the business. Other resources such as data scientists, enterprise or solutions architect, data annotation specialist and project owner can be outsourced. It is important to recruit resources that tackle a variety of data science problems – from structured data to unstructured documents and images. Both the breadth and depth of skills and knowledge are important. A good approach is to start small, think big: Applications of AI are everywhere within an organization, and the potential of this technology will only increase with time. I suggest selecting use cases that are most impactful to the business and getting started with a modest approach on hiring resources, but with a business vision and an expansion strategy in mind in full alignment with the company’s mission.
Sign up for the free insideBIGDATA newsletter.
Speak Your Mind