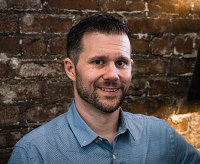
In this special guest feature, Duncan Curtis, Vice President of Product Management at Samasource, believes that contrary to popular understanding, the key to AI’s success is the combination of human oversight and skillfully trained data, not pure automation. Duncan brings 3 years of Autonomous Vehicle experience as the Head of Product at Zoox (now part of Amazon), and 4 years of AI experience from his product management days at Google where he delighted the +1B daily active users of the Play Store and Play Games. Prior to this, Duncan’s career was focused on mobile gaming, most notably working on the Fruit Ninja and Jetpack Joyride franchises. Duncan studied Computer Software Engineering at Queensland University of Technology. He is excited to bring his love of technology and impact together at Samasource.
Since 2015, enterprise use of AI-powered technologies has increased by 270% globally. Today, more than 85% of Americans use these products on a daily basis. While these innovations are already improving efficiencies and opening doors to new technologies like autonomous vehicles and virtual assistants, the conveniences they afford us can be exploited if not guided appropriately.
With AI spending projected to hit $97.9B by 2023, we’re at a pivotal moment in establishing the precedent for future innovations. While it may seem that the industry is striving for complete automation, AI requires the guiding hand of trained human specialists. In fact, without successfully trained data and expert, diverse human oversight, the future of AI has the potential to cause more harm than good.
The Foundation of Successful AI
Training data is an essential foundation for ensuring AI projects fulfill their promises. AI systems from Sophia the Robot to Siri to Tesla, are fed data to develop and refine their machine learning algorithms, but if the information used to teach the program is not diverse and robust, the program itself will not act properly. In other words: quality in equals quality out.
Data training is a process through which human annotators detect, select, and label objects across image, video and 3D model renderings. AI algorithms then process this annotated data and use it to make informed decisions.
Despite the concept’s simplicity, 96% of AI programs run into problems with data quality, and 8 out of 10 of these projects fail outright. Beyond creating an inefficient product, untrained data can result in dangerous biases that inform critical decisions in industries spanning healthcare, conservation, autonomous vehicles and VR.
These vulnerabilities are more common than many providers would like to admit. In 2012, doctors at Memorial Sloan Kettering Cancer Center leveraged IBM’s Watson AI to inform critical diagnosis and treatment decisions. However, the solution’s foundation of hypothetical patient data was found ineffective when it produced unsafe recommendations. In 2017, Amazon’s Alexa came into question when a television ad triggered its purchase of several dollhouses. More recently, Robert Julian-Borchak Williams was wrongfully accused and arrested on a charge of larceny by a Michigan facial recognition system.
These disparate errors are caused by the same inherent flaw: incomplete training data. The high-tech hype surrounding the technology often blinds AI providers from acknowledging the need for human intervention to ensure errors do not persist. While relying on smaller, less diverse data sets can save time and money, these shortcuts create structural flaws that threaten the success of the AI.
Why AI Requires Humans-in-the-Loop
Despite a public dialogue that’s primarily concerned with AI usurping humans, the technology cannot function without human oversight. In fact, AI is only reliable and therefore, successful when it combines its advanced technological capabilities with a diverse, expert human-in-the-loop team.
In the data training process, humans are responsible for labeling varied datasets. Within the autonomous vehicle industry, for example, human-in-the-loop teams are presented with visual assets of a series of streets, freeways and intersections. Each annotator then selects and labels the objects in the imagery such as pedestrians, street signs and motorcycles, training the machine learning algorithms to later detect and comprehend these objects in the future. With only AI labeling the initial data set, important objects may be missed, leading to AI ‘drivers’ that miss pedestrians in the real world.
Humans are not only necessary for labeling data correctly, but in creating the datasets themselves. If there is no human oversight of the contents of a training data set, then bias may also be introduced at this preliminary stage. Imagine the potential vulnerabilities of an autonomous vehicle that contained no snow driving in its training data set and later encountered it in the real world.
Until we reach the Singularity, pure autonomy is not a feasible possibility because AI training and operation requires human oversight. The success of AI decision-making depends primarily on the skill of the human team trained to provide high-quality annotation and model validation. Similarly to how we expect a certain degree of pedigree from those in positions of power, why should we allow untrained AI to make critical decisions across healthcare, criminal justice, public policy, and more?
Contrary to popular understanding, the key to AI’s success is the combination of human oversight and skillfully trained data, not pure automation. As AI becomes more accessible and prevalent to our daily lives, the practices we instill now will inform the effectiveness of our future technology. By ensuring that data sets are high-quality and their AI works in tandem with a diverse human-in-the-loop-team, providers can be confident that their solutions will succeed.
Sign up for the free insideBIGDATA newsletter.
Speak Your Mind