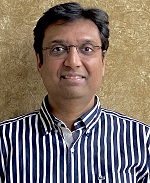
In this special guest feature, Vatsal Ghiya, CEO and co-founder of Shaip, explores the three factors that he believes will allow data-driven AI to reach its full potential in the future: the talent and resources necessary to construct innovative algorithms, an immense amount of data to accurately train those algorithms, and ample processing power to effectively mine that data. Vatsal is a serial entrepreneur with more than 20 years of experience in healthcare AI software and services. Shaip enables the on-demand scaling of its platform, processes, and people for companies with the most demanding machine learning and artificial intelligence initiatives.
The term “artificial intelligence” was first used in 1955, but there’s a reason we’re only now beginning to enter the golden age of this technology. AI requires three main ingredients to reach its potential: the talent and resources necessary to construct innovative algorithms, an immense amount of data to accurately train those algorithms, and ample processing power to effectively mine that data.
Today, that triumvirate has been firmly established, and these individual factors are propelling AI to heights that are difficult to imagine from our current vantage point.
AI Gains Altitude
Many experts believe that AI will bring about a Fourth Industrial Revolution, and the past few years have seen organizations around the world jockeying for position on the cutting edge of the transition. Data from CB Insights reveals that AI startups raised a record $26.6 billion in 2019, with areas such as autonomous driving and facial recognition software generating the biggest buzz.
These startups are also aggressively pursuing tech talent in AI and data science, and these fields will only continue to grow as a result. Despite the increased competition over talent, the gap between research and implementation of AI has shrunk due to the growing suite of implementation tools and open-source communities that have emerged around the technology.
Even the most brilliant technologists in the world need data to accurately train an AI engine, and the Internet of Things is ready to provide it. Statista estimates that there are about 31 billion installed IoT devices today, meaning data isn’t just accessible — it’s unavoidable. While the organizations that can’t properly put data to use are virtually drowning in it, AI leaders are busy training algorithms on these billions of data points generated at the intersection of consumer and machine interaction.
Of course, billions of data points place serious demands on computing equipment, but the proliferation of cloud computing and its constantly dwindling cost have enabled businesses of all sizes to access the resources they need. Data from Right Scale’s 2019 State of the Cloud Report found that 91% of businesses utilized the public cloud, while 72% opted for a private one. Besides the computing capabilities offered by IaaS, SaaS, and PaaS cloud services, the cloud also encourages collaboration across teams that will empower additional AI development — even when the teams’ members are scattered around the world.
Anticipating the AI Future
Thirty years ago, computer vision systems could barely recognize handwritten digits. Now, AI is powering self-driving vehicles, detecting malignant tumors, and scanning legal contracts to make sure they’re airtight. Conversational AI is another exciting area, and the audio captured by devices such as Amazon’s Alexa and Google Home is informing future customer-support tools, computer accessibility software, and even healthcare services. Along with advanced algorithms and powerful computing resources, accurately labeled datasets play a key role in AI’s renaissance.
According to the Cognilytica Data Annotation 2019 report, the $150 million market for third-party data labeling solutions in 2018 will have grown to more than $1 billion by 2023, and for every dollar spent on these solutions, two more will go toward internal efforts to support them. While the report anticipated that machine learning-augmented intelligence would be a core part of data preparation tools by 2021, it also found that humans would necessarily remain in the loop for quality control purposes.
Any AI implementation will hinge on the availability of data, but not any data will suffice. It’s often said in the industry that “garbage in means garbage out.” As we watch the future of AI unfold, it will be critical to remain on the lookout for bias in AI that produces undesirable results. The three converging trends of growing investment, ubiquitous data availability, and cheap cloud computing power will produce the next generation of AI tools, but whether those tools can achieve ideal outcomes depends on the quality of data they were trained with.
Sign up for the free insideBIGDATA newsletter.
Join us on Twitter: @InsideBigData1 – https://twitter.com/InsideBigData1
Speak Your Mind