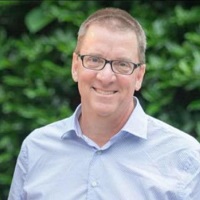
In this special guest feature, Jerry Kurtz, EVP of Insights & Data at Capgemini North America, discusses how laying the right foundation early is essential to scale AI programs in the long-term. He provides some key examples of important areas AI leaders should prioritize when kicking off their AI programs to ensure they are positioned to scale in the future. Jerry has more than 30 years of management consulting experience working primarily in the manufacturing, high-tech, consumer products, retail, and logistics industries. His leadership experience includes data & analytics, artificial intelligence, internet of things, enterprise transformation with large scale ERP, supply chain management, shared services and business process services. Jerry lives in Charlotte, N.C., and received his Bachelor of Engineering degree from Vanderbilt University.
There are multiple paths to every destination. For example, driving your car may result in less distance traveled, but taking the train can bypass potential traffic. A trans-continental flight has proven to save more time than traveling by boat.
AI leaders want to grow and scale their programs, but how they scale them in the most efficient and effective way – and choose the right starting point and sequenced roadmap – is the challenge. Research shows that only 13% of organizations have successfully scaled their AI programs beyond the pilot and initial production stages. During the pandemic, these were the companies who were able to continue progressing their AI transformations while many of the others put their programs on pause.
To achieve true scalability, AI programs must be planned well from the start, with a solid, value-based roadmap in place to set the program up for massive success. For simplicity’s sake, let’s refer to this preliminary stage of work as “Phase Zero.” When mistakes are made in Phase Zero, it becomes much harder to earn buy-in from the business to keep moving the program forward. There is no one-size-fits-all approach: each unique roadmap and business case is dependent on the organization’s customized needs and circumstances. There could be gaps in data foundation and AI strategy, or there could be missing critical components in the data governance and/or innovation pillars. The key is to assess the situation thoroughly and create the appropriate target state + roadmap that leads to AI at scale and does so in a way that maximizes value to the business over time, with ROI coming early and often.
Here are some key examples of important areas AI leaders should prioritize when kicking off their AI programs to ensure they are positioned to scale in the future:
High impact use cases
There is simply no substitute for quick wins that provide quick value to the business. By quick wins, we are referring to business use cases that have high ROI with quick payback. This means that costs are relatively low while value of solving the business use case is high. In addition to these financial considerations, these quick wins will almost always have the right underlying data readily available to solve the problem. If they don’t, then the projects will typically take longer and have lower payback, with data transformation being on the critical path. These areas of “low-hanging fruit,” if done correctly, may also lead the organization to start seeing AI’s full potential once it scales. Quick wins are typically chosen because they also have a senior executive business sponsor who is ready to help the team overcome any business obstacles that may stand in the way of success.
A client may want a quick and easy way to provide a more immersive on-board digital experience. Drawing on data science and machine learning techniques to enable personalization, it’s possible to help the organization to transform its customer 360, data quality and segmentation all through an innovative, AI-enabled approach. This may include a large-scale Data Cloud designed and built to collect, process, and analyze everything from products and pricing to advanced marketing data. AI can be used to detect and improve data quality, and an AI based recommendation engine can be deployed to allow personalization at scale for marketing and sales across multiple channels. By focusing on a targeted and impactful use case, the company be enabled to re-shape the customer experience with better quality data and by customized and personalized offers and product recommendations.
More on low cost, high yield projects
When strategizing the right path forward and laying the groundwork, it’s vital to prevent cost from being a barrier. In most phase zero efforts, we typically identify projects that will cost less up front, but still provide quick financial returns. By doing so, these programs gain momentum that can also help to “self-fund” other, more challenging, and transformational efforts which will be required to scale to dozens of use cases.
For example, a telecommunications company might realize they were only spending 15% of their analytics budget on operations. Through analysis, it could be discovered there are efficiency gaps across the ecosystem as the organization was operating in silos. By creating a roadmap and simplifying to a cloud data platform, the organization could be enabled to decrease its number of applications. This optimization could result in ROI to save the company significant costs over the following years.
Equip teams with the right resources
The best plans still need the right resources and teams to execute them, and there is an undisputed shortage of talent in the data, analytics, and AI spaces in the world today. Discovering any gaps in training, tools, or technology capabilities and assessing how to fill them is imperative at these early stages of your AI journey. By doing so, teams can get the resources they need when they need them – setting them up to start building the fundamental strongholds of a successful AI program.
To experience the true benefits of AI, programs must clear the hurdles that prevent so many from achieving AI at scale. This starts with a strategic roadmap that is built properly and includes the most logical sequence and steps that align to the individual company’s situation and business objectives. With the right selection of early business use cases, and by setting teams up for success with the resources they need to execute, AI leaders can reach scale much faster than previously imagined. The ongoing, underlying data transformation must proceed in parallel, but can be partially funded by the value from the business use cases if the roadmap is properly designed. There is simply no better money spent in the data, analytics, and AI space than the small investment required to build a roadmap that provides you with the best ROI, payback, and scale over time.
Sign up for the free insideBIGDATA newsletter.
Join us on Twitter: @InsideBigData1 – https://twitter.com/InsideBigData1
Speak Your Mind