It’s estimated that some 70% of the business applications companies use are SaaS-based – and that percentage continues to grow. While this has undoubtedly improved efficiency, productivity and collaboration for many companies, it’s also created an expanded attack surface and new entry points. For many organizations, there’s a lack of overall visibility into the users and data for the SaaS applications used. And that’s scary, because it’s almost impossible to secure and protect what you can’t see (and may not be aware of.)
IT teams need a way to enforce security policies and ensure that sensitive data isn’t being inappropriately shared via these tools. And they need to be able to do this without having a significant impact on efficiency and productivity.
It might be tempting to try and address SaaS security concerns by simply applying “automation” and setting some overarching rules, but the problem with this approach is that sometimes you throw out the baby with the bathwater. In other words, you can wind up preventing employees from doing work or sharing sensitive information that they need to do. This is where the idea of self-supervised learning can help apply policies and rules with context.
The challenges of a “set it and forget it” approach
Automation is key to addressing the challenge of securing SaaS data, but it can’t be done without the proper context.
Let’s say you set it up so that your system will automatically prevent or block any exchange involving sensitive information. Here’s the problem with that approach: Most of the time when someone shares sensitive information, it’s necessary to fulfill their job role – especially if they’re working in a function such as human resources or finance that deals with a lot of sensitive information. A workflow that automatically prevents sensitive information being shared could end up having a significant impact on these departments’ ability to do their work.
Even if you take a more measured approach – let’s say you set it up so that sensitive information can be shared, but users will lose access after a specific period of time – that can also have a negative impact on workflow and productivity. And it still doesn’t solve the problem of protecting access to that sensitive information in the first place.
Automation isn’t something that can just be broadly applied in a blanket manner when it comes to SaaS tools and data. What you need is context.
Creating a more contextualized process
Context applied to automation can help decrease risk and address issues without introducing unnecessary friction. It’s a way to find a happy medium between business goals and security goals.
When evaluating an action to determine whether it is appropriate, context refers to the capacity to comprehend the larger ecosystem. With this information in hand, a security team can determine who collaborates with whom; determine who has permission to use particular tools, systems or data; and decide whether a given activity is appropriate.
With a self-supervised learning approach, the model can train itself using the data it has been given. It doesn’t require explicit labels or instructions from humans. Self-supervised learning can be used to analyze the connections between employees and understand the patterns of communication and collaboration within a company. The model can learn about the normal behavior and identify any abnormal or anomalous behavior, which can be used to improve security and protect sensitive information. Additionally, it can also help to provide a more accurate and efficient way of mapping sensitive data.
Getting started with self-supervised learning
With self-supervised learning, you can gain an understanding of the organizational context and mapping sensitive data based on that context. This allows the algorithm to learn what normal or good behavior looks like and identify any abnormal or anomalous behavior that could indicate a security risk.
To start, you will need to connect your main collaboration tools via an API, such as O365, Slack, Github, or GoogleWorkspace. Self-supervised learning’s advanced analytics will crunch the history data and use it to train the algorithm. Once the algorithm has been trained, you can begin using it to monitor your organization’s activities and identify potential security risks. The algorithm will continuously learn and adapt.
Once the self-supervised model has a good understanding of your organizational context, it will allow you to configure policies and workflows that are specific to your organization. Furthermore, you’ll be able to use that information to add context to your existing policies and automated workflows.
Another benefit is that the analytics will automatically detect exposure of sensitive data based on the context learned. A security analyst’s role will be to monitor the output of the self-supervised learning system, and to make any necessary adjustments to the data or business policies and actions that it generates.
Augmenting human intelligence
It is important to keep in mind that self-supervised learning is not a replacement for human oversight and analysis. Security analysts should regularly review the model’s output and use their own expertise to make final decisions on security policies and automation.
To get the best results, it’s important to work closely with experts in the associated business groups and security to ensure that the model is properly configured and implemented. This will allow you to get the most out of your security applications and protect your organization’s sensitive data.
With the right approach, your self-supervised learning analytics can be a powerful tool for securing SaaS applications. When configuring automation and policies based on a context generated by self-supervised learning, it’s important to start by understanding your organization’s unique needs as not every organization is the same. This includes identifying the sensitive data that needs to be protected and understanding the typical behavior of users on an organizational social network graph.
Additionally, it’s important to be transparent about how the model is making decisions and to ensure that it is explainable and trustworthy.
Self-supervised for SaaS security
SaaS tools and apps have revolutionized the workplace in many ways – and we certainly don’t want to put an end to that. But organizations do need to think carefully about the security implications of these apps and the sensitive data that’s being shared within and across them. An approach based on self-supervised learning can help improve your organization’s security posture by providing a more agile and context-aware approach to security. The ability of the system to continuously learn and adapt to the changing environment of your organization, helps to identify and handle security threats in a more efficient and effective way while facilitating the normal day-to-day business functions.
About the Author
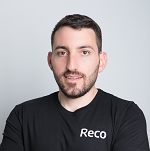
Tal Shapira, Ph.D., is the co-founder and chief scientist at Reco, a collaboration security company that delivers unprecedented visibility, detection and control over information shared in collaboration platforms like Slack, Microsoft 365 and Google Workspace. He is a graduate of the Taipot Excellence Program, with an in-depth knowledge of data science, deep learning, big data and cybersecurity R&D, with a demonstrated history of working in the military industry. He was previously head of cybersecurity group within the Israeli prime minister’s office and served as head of algorithms at Guardian Optical Technologies, which was acquired by Gentex.
Sign up for the free insideBIGDATA newsletter.
Join us on Twitter: https://twitter.com/InsideBigData1
Join us on LinkedIn: https://www.linkedin.com/company/insidebigdata/
Join us on Facebook: https://www.facebook.com/insideBIGDATANOW
Speak Your Mind