Generative AI adoption is a critical initiative for enterprises across banking, insurance, and manufacturing today. The technology provides the potential for leaders to build a new organization that not only complements the existing business but also over time allows them to fundamentally transform the business itself. At the simplest level, organizations can streamline and automate simple tasks and complex processes with it. Without it, organizations risk losing their ability to compete with those that have adopted and use it.
While it is a newly developing technology, like any other enterprise IT initiative, the adoption of generative AI requires vision, analysis, planning, testing, and measurement to ensure the fastest and smoothest deployment and to derive maximum value. It also necessitates a framework for adoption.
An adoption framework can make the AI work
A generative AI enterprise adoption framework entails an end-to-end journey encompassing vision, goals, desired outcomes, and return on investment. Enterprises should commence with vision and ideation, involving the identification of use cases and business processes where AI implementation is required. Subsequently, proceed to the execution stage, wherein applications are developed based on the ideations and shortlisted use cases. Following this, thorough testing, deployment, monitoring, and measurement of results should be conducted.
If in-house skills are lacking or supplementation is needed, consider engaging experts and consultants with experience who can guide at every step, facilitating skills transfer for self-sustainment.
Although for many enterprise business and IT journeys, such as digital transformation typically take several years to deploy and yield results, the use case model of generative AI deployments can be implemented and yield results in months, while a comprehensive enterprise-wide transformation will require years. Within the enterprise adoption framework for generative AI, it is best to divide the adoption journey into multiple horizons, such as H1, H2, and H3, or stages, based on complexity and time. Each horizon can be a project or group of projects with defined objectives, timelines, and stages.
Generative AI enterprise deployments in banking and insurance may take longer because these industries have to manage the regulatory framework and ensure that they are protecting customers’ private financial and personal data.
The categories of generative AI and how they fit into an enterprise adoption framework
In addition to creating horizons as part of an enterprise adoption framework for generative AI, it’s important to recognize two categories of generative AI and organize accordingly. The two categories are everyday AI, also called assistive AI, and transformational AI, also referred to as augmented or autonomous AI.
Everyday AI, as the name suggests, is designed for enhancing internal and external task productivity. Examples of everyday AI include website chatbots for customer engagement and the use of AI to sift through documentation on how to use a product for internal or external support. Other examples include IT asset discovery and documentation, which assist organizations in improving cybersecurity, preparing for modernization and digital transformation, and assessing regulatory compliance. Software developers now leverage everyday AI for optimal efficiency in coding and debugging.
Transformational AI applies to business processes, such as integrating procurement, finance and accounting systems to streamline the interaction between procurement for ordering goods and services, and finance for authorization, payment and accounting. Another example of transformational AI is fraud detection, where AI can monitor systems for anomalies and suspicious behavioral patterns exponentially better than humans, and generate alerts and visualizations for human review, insight and action. Transformational AI also applies to automating business processes and emulating human intelligence, such as evaluating and scoring the risk of a new insurance policy, investment or loan.
The two categories of AI can also be mapped to the horizons of an enterprise adoption framework for generative AI. H1 horizons can cover simpler, shorter-term projects involving everyday assistive AI and quick wins, completing them within three to six months. H2 horizons involve augmenting business processes with transformational AI while keeping humans in the loop; this phase can span six months to one year. H3 horizons are designated for automating business processes with transformational AI and typically last one to three years or longer.
Beyond the framework: Generative AI adoption challenges
To create a framework for implementing the use cases, goals, categories, workstreams, and timeframes of generative AI adoption, organizations need to address challenges and issues related to human resources, culture, governance, responsible AI, and regulatory compliance. This ensures efficient and smooth generative AI adoption.
Organizations need to consider and plan for how the adoption of generative AI will impact jobs. They must assess whether jobs will be eliminated or created, determine if affected employees can be reassigned, and decide whether hiring will be necessary for the success of generative AI. Additionally, the adoption of generative AI will also influence organizational culture. The way in which an organization and its employees work will undergo changes, making communication and change management initiatives crucial to facilitate smooth adoption.
Furthermore, organizations must optimize AI governance and deploy responsible AI practices. This involves addressing potential pitfalls, such as bias and hallucinations, and ensuring the accuracy of the output from AI models.
Responsible AI also encompasses cybersecurity, regulatory compliance, and data privacy. Organizations must ensure that, like all their information systems, their generative AI applications and systems comply with all applicable laws and regulations, such as the General Data Protection Regulation in Europe, the California Consumer Privacy Act, and laws stemming from President Biden’s Executive Order on Safe, Secure, and Trustworthy Artificial Intelligence.
The rapidly evolving landscape of generative AI innovations and regulations is causing many organizations, especially in the banking, insurance, and manufacturing industries, to proceed with generative AI adoption and live deployment slowly and cautiously. However, there are workarounds that enable progress while organizations wait and watch. For example, organizations can and should use synthetic data with the same structure and volume as their production data to test their generative AI systems for performance, cybersecurity, data privacy, and regulatory compliance. Once ready, organizations can then move to live deployments with their production data without losing ground to their competition.
By employing an enterprise adoption framework for generative AI and addressing challenges and issues related to human resources, culture, governance, responsible AI, and regulatory compliance, organizations can efficiently and seamlessly adopt generative AI, enabling them to take giant strides towards digital transformation and becoming an AI-first organization.
About the Author
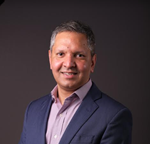
Ajay Kumar, CEO at SLK Software. Ajay Kumar is a passionate leader known for his positive client impact, driving revenue growth and market expansion and an extensive experience in managing P&Ls across all verticals. An industry veteran, Ajay’s entrepreneurial spirit is evident in driving digital transformation and growth strategies across various industries.
Ajay’s leadership philosophy centers on the power of collaboration & empowerment, fostering a culture of innovation and inclusivity, inspiring creative thinking and challenging status quo. As the CEO of SLK Software, Ajay brings a wealth of experience and expertise in intelligent business transformation, automation, digital innovation, and AI. Throughout his career, Ajay has held leadership positions where he consistently delivered successful outcomes, led global business units and built high-performance teams.
In addition to his engineering master’s degree, Ajay holds an MBA from IIM Bangalore and has completed an Executive Education program at Stanford University.
Sign up for the free insideBIGDATA newsletter.
Join us on Twitter: https://twitter.com/InsideBigData1
Join us on LinkedIn: https://www.linkedin.com/company/insidebigdata/
Join us on Facebook: https://www.facebook.com/insideBIGDATANOW
Speak Your Mind