The computer chip industry over the last couple of decades has seen its innovation stem from just a few top players like Intel, AMD, NVIDIA, and Qualcomm. In this same time span, the VC industry has shown waning interest in start-up companies that made computer chips. The risk was just too great; how could a start-up compete with a behemoth like Intel which made the CPUs that operated more than 80% of the world’s PCs? In areas that that Intel wasn’t the dominate force, companies like Qualcomm and NVIDIA were a force for the smartphone and gaming markets.
The recent resurgence of the field of artificial intelligence (AI) has upended this status quo. It turns out that AI benefits from specific types of processors that perform operations in parallel, and this fact opens up tremendous opportunities for newcomers. The question is – are we seeing the start of a Cambrian Explosion of start-up companies designing specialized AI chips? If so, the explosion would be akin to the sudden proliferation of PC and hard-drive makers in the 1980s. While many of these companies are small, and not all will survive, they may have the power to fuel a period of rapid technological change.
There is a growing class of start-ups looking to attack the problem of making AI operations faster and more efficient by reconsidering the actual substrate where computation takes place. The graphics processing unit (GPU) has become increasingly popular among developers for its ability to handle the kinds of mathematics used in deep learning algorithms (like linear algebra) in a very speedy fashion. Some start-ups look to create a new platform from scratch, all the way down to the hardware, that is optimized specifically for AI operations. The hope is that by doing that, it will be able to outclass a GPU in terms of speed, power usage, and even potentially the actual size of the chip.
Accelerated Evolution of AI Chip Start-ups
One of the start-ups that is well-positioned to enter the battlefield with the giant chip makers is Cerebras Systems. Not much is known publicly about that nature of the chip the Los Altos-based startup is building. But the company has quietly amassed a large war chest to help it fund the capital-intensive business of building chips. In three rounds of funding, Cerebras has raised $112 million, and its valuation has soared to a whopping $860 million. Founded in 2016, with the help of 5 Nervana engineers, Cerebras is chock full of pedigreed chip industry veterans. Cerebras co-founder and CEO Andrew Feldman previously founded SeaMicro, a maker of low-power servers that AMD acquired for $334 million in 2012. After the acquisition, Feldman spent two and a half years as a corporate vice president for AMD. He then started up Cerebras along with other former colleagues from his SeaMicro and AMD days. Cerebras is still in stealth mode and has yet to release a product. Those familiar with the company say its hardware will be tailor-made for “training” deep learning algorithms. Training typically analyzes giant data sets and requires massive amounts of compute resources.
Another massive financing round for an AI chip company is attributed to Palo Alto-based SambaNova Systems, a startup founded by a pair of Stanford professors and a veteran chip company executive, to build out the next generation of hardware to supercharge AI-powered applications. SambaNova announced it has raised a sizable $56 million series A financing round led by GV, with participation from Redline Capital and Atlantic Bridge Ventures. SambaNova is the product of technology from Kunle Olukotun and Chris Ré, two professors at Stanford, and led by former SVP of development Rodrigo Liang, who was also a VP at Sun for almost 8 years.
A number of other AI chip start-ups are working to design next-generation chips with a multitude of computing cores targeting the kind of parallelizable mathematics upon which deep learning algorithms are based. Campbell, California-based startup Wave Computing recently revealed details of its Dataflow Processing Unit (DPU) architecture that contains more than 16,000 processing elements per chip. The benefits of Wave Computing’s dataflow-based solutions include fast and easy neural network development and deployment using frameworks such as Keras and TensorFlow. Additionally, Bristol, England-based start-up Graphcore said its chip will have more than 1,000 cores in its Intelligence Processing Unit (IPU). Wave Computing has raised $117 million and Graphcore has raised $60 million.
Chinese start-up Cambricon recently raised $100 million in a Series A round led by a Chinese government fund for its AI chip development. Another Beijing chip start-up, DeePhi, has raised $40 million, and the country’s Ministry of Science and Technology has explicitly called for the production of Chinese chips that challenge NVIDIA’s.
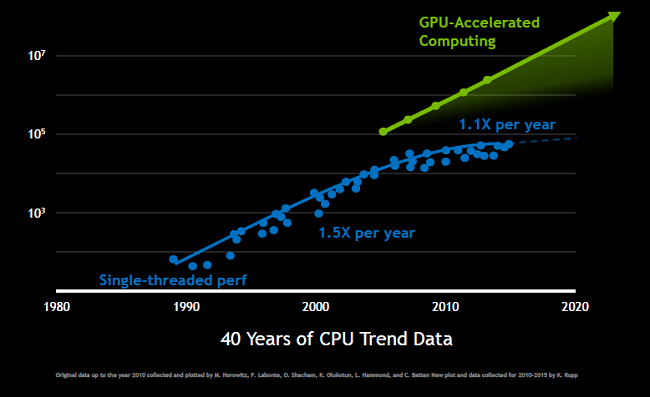
Courtesy of NVIDIA
While these levels of funding might sound massive, it’s becoming a pretty standard amount of investment for start-ups looking to cultivate this space. It opens an opportunity to beat massive chip makers and create a new generation of hardware that will be ubiquitous in running devices that are built around AI. These AI chip start-ups believe they can build chips that outperform GPUs in deep learning applications (GPUs have far more cores than CPUs). Indeed, the money has been pouring in. Anticipating the prospect of taking on NVIDIA, which has a market cap of nearly $100 billion (up nearly three fold in the past year), VCs have loosened their wallets for these bold new AI chip makers.
Despite all the excitement, there are numerous challenges. For one, none of the new hardware is out yet. The process of developing a chip can take years, and for now much of the hardware remains in development or staged in early pilot programs. As a result, it’s hard to anticipate which of the start-ups will get on a path to deliver on their promises.
Another challenge centers around the difficulty of staying an independent chip company with M&A activity reaching a frenetic pace. The past few years have seen the semiconductor industry go through waves of consolidation as chip giants search for the next big evolutionary step. Most of the acquisitions have targeted specialized companies focused on AI computing with applications like autonomous vehicles. For example, industry mainstay Intel has been the most aggressive, paying $16.7 billion for programmable chipmaker Altera in 2015, and $15 billion for driver assistance company Mobileye in 2017. In addition, in 2016 Intel acquired Nervana, a 50-employee Silicon Valley start-up that had started building an AI chip from scratch, for $400 million.
Qualcomm is currently making a bid for NXP, a chipmaker with a big presence in the auto market.
Big Players Executing on Major Pivots
For now, the market for deep learning chips is overwhelmingly dominated by NVIDIA. The company has long been known for its GPUs, which have been widely used in games and other graphically-intensive applications. A GPU has thousands of processors operating in parallel. These “cores” typically perform lots of low-level mathematical operations to perform tasks like image classification, facial recognition, language translation and much more. A few years ago, researchers discovered that the capabilities of GPUs were ideally suited to run deep learning algorithms, which require thousands of parallel computations. The discovery put NVIDIA at the heart of the AI revolution.
Google has already developed its own AI-focused chips called the Tensor Processing Unit (TPU), whereas Microsoft seems committed to using a chip called field-programmable gate arrays (FPGA). Recently, Google said it would allow other companies to buy access to its TPU chips through its cloud-computer service. Google hopes to build a new business around the TPUs. Google’s move highlights several sweeping changes in the way modern technology is built and operated. Google is the vanguard of a worldwide movement to design chips specifically for AI. TPU chips have helped accelerate the development everything from the Google Assistant, the service that recognizes voice commands on Android phones, to Google Translate, the internet app that translates one language into another. There’s a common thread that connects Google services such as Google Search, Street View, Google Photos and Google Translate: they all use Google’s TPU to accelerate their neural network computations behind the scenes. They are also reducing Google’s dependence on chip makers like NVIDIA and Intel. In a similar move, Google designed its own servers and networking hardware, reducing its dependence on hardware makers like Dell, HP, and Cisco.
In addition, a published report from The Information states that Amazon is developing its own AI chip designed to work on the Echo and other hardware powered by Amazon’s Alexa virtual assistant. The chip reportedly will help its voice-enabled products handle tasks more efficiently by enabling processing to take place locally at the edge, by the device, rather than in AWS.
What’s Ahead
It is with caution that any of these new companies fantasize about challenging Intel head-on with their own chip factories, which can take billions of dollars to build. Start-ups tend to contract with other companies to make their chips. But in designing chips that can provide the particular kind of computing power needed by machines learning how to do more and more things, these start-ups are racing toward one of two goals: find a profitable market niche or quickly get acquired. Because of the upsurge in demand for a new kind of compute resource that drives deep learning, many believe this is one of those rare opportunities when start-ups have a chance to give the entrenched giants a run for their money.
Contributed by Daniel D. Gutierrez, Managing Editor and Resident Data Scientist at insideBIGDATA. In addition to being a tech journalist, Daniel also is a practicing data scientist, author, educator and sits on a number of advisory boards for various start-up companies.
Sign up for the free insideBIGDATA newsletter.
No mention of IBM?
Yes! Some exciting IBM news was announced at the NVIDIA GTC conference today: https://www.ibm.com/blogs/systems/memory-matters-AI/
are any of these companies listed on the stock exchanges?