Perhaps the lone certainty in today’s tenuous business climate is that, whatever else may come tomorrow, the burgeoning data landscape will continue its march towards more and more decentralization.
The uncharted growth of the cloud, edge computing, the Internet of Things, and the remote work paradigm easily reveal as much.
Although these developments are terrific for distributed collaborations and a consummate view of customers while reducing the latency of information for intelligent decision-making, they have very real ramifications for the fundamentals of data management—not all of which are as rosy.
For many pragmatic use cases (like simply querying data, performing data discovery, and engineering data for application or analytics consumption), the growing distribution of the data landscape simply reinforces the need for centralization. Most organizations respond by replicating data between locations which, although providing some short term viability, isn’t truly sustainable.
“We can’t just keep moving and copying data in order to manage it,” warned Stardog CEO Kendall Clark. “There’s an endpoint where that just doesn’t work anymore and generally, we’re closer to that point than people realize.”
Data virtualization has arisen as a dependable alternative to endlessly replicating data and incurring the woes this method produces. When properly implemented with modern data models, it creates two benefits that solve the riddle of ‘centralizing’ decentralized data by enabling users to “leave data within existing data sources and perform all these complex queries where the data lives, on-prem or in the cloud,” Clark affirmed.
Thus, without data ever moving, organizations can access them in a centralized fashion for a number of gains in data quality, data integration, and other pillars of data management.
Data Quality
One of the paramount reasons constantly copying data is untenable is this practice’s noxious impact on data quality. Replicating data between locations can reinforce data silos and raise questions about which versions are correct—which inevitably happens after manipulating data downstream in different places. In this instance, the particular use case determines the data quality ‘standards’, which aren’t always trustable when using those data for more than one application or more than one time.
“The data copying or data movement that I’m concerned about is when we make copies and everyone can see all the different copies,” Clark commented. “Not everyone, but when they’re visible to the organization. That brings on issues like which one is current, which one are we updating? It causes confusion.” This situation is readily ameliorated by the abstraction layer data virtualization provides, in which data remain where they are but are accessed via a centralized platform. With the other approach, companies risk “up to date data, data currency, data staleness, and data freshness issues,” Clark indicated.
Schema
Another pivotal distinction of virtualization technologies is that data—as described in data models—are effectively liberated from their storage layer. This supports data management benefits like reusable schema for modeling data for a sundry of use cases, as opposed to tying down data models to specific applications.
Such reusability is advantageous for expediting aspects of data preparation and decreasing the time to action for data-driven processes. “In this regard, what powers the virtualization capability from the user’s point of view is that same business level meaning and context data modeling,” Clark observed. This capability is substantially improved by relying on universal data model standards characteristic of semantic graphs.
Data Integration
The fundamental benefit of this aspect of virtualization is data integration, which is more important than ever with the surplus of heterogeneous data sources outside the enterprise, many of which involve structured and unstructured data. “If the integration and connection [of data] exist only at the physical layer, then changes at the physical layer break the integrations—or they can,” Clark commented. “All we’re trying to do is uplevel the game and make there be another place where you can do the integration and the connecting that’s abstracted from the storage.”
Therefore, organizations can move data (if they want to) wherever it makes the most sense, such as next to where the compute occurs for time-sensitive use cases in the cloud, perhaps. “This is a good thing now, because now the storage level can evolve independently,” Clark remarked. “That’s a good thing for the bottom line.” Most of all, when organizations do want to move data, they can do so “without breaking things,” Clark mentioned—or spending lengthy periods of time recalibrating data models, working on integrations, and delaying time to value.
Unstructured and Semi-Structured Data
The utilitarian nature of standards-based data models complements the universal accessibility organizations achieve with data virtualization. Semantic graph models are ideal for conforming even unwieldy semi-structured and unstructured data to the same schema used for structured data. By leveraging this model to buttress data virtualization capabilities, “The benefit to adding graph to the virtualization story is the ability to virtualize or connect over a bigger percentage of the enterprise data landscape that matters,” Clark revealed. “We’re just not in a world anymore where it’s just about relational data.”
The virtualization of semi-structured data alongside structured data makes both equally accessible to enterprise users. Moreover, the data virtualization approach eliminates the need to even conceive of data in these terms, particularly with the standards-based approach to data modeling true knowledge graphs utilize. “The key benefit to bringing graph and virtualization together from the customer point of view is you can just get at more of the data,” Clark summarized.
The Chief Value Proposition
The increasingly distributed nature of the data landscape signifies many things. It’s a reflection of the remote collaborations characteristic of working from home, the takeoff of the cloud as the de facto means of deploying applications, and the shift to external sources of unstructured and semi-structured data. However, it also emphasizes issues pertaining to data quality, schema, and data integrations that are foundational to data management.
Data virtualization enables organizations to surmount the latter obstacles to focus on the former benefits. Supplementing it with mutable graph data models boosts its applicability to data of all types so companies can confidently “query data where it lives, without moving or copying it,” Clark explained. “If you had to summarize the value proposition in a little bit of an abstract way…the primary one is querying data to drive some business outcome without having to move or copy the data that’s relevant to that business question.”
About the Author
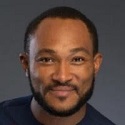
Jelani Harper is an editorial consultant servicing the information technology market. He specializes in data-driven applications focused on semantic technologies, data governance and analytics.
Sign up for the free insideBIGDATA newsletter.
Join us on Twitter: @InsideBigData1 – https://twitter.com/InsideBigData1
Speak Your Mind