Whether in the form of Robotic Process Automation, chatbots, or some other type of digital assistants, the presence of intelligent bots is substantially increasing across the data ecosystem … in more ways than one.
The diversification of the number of tasks these bots can perform is multiplying, as is the intrinsic complexity of those jobs, which unambiguously benefits knowledge workers worldwide.
Whether dynamically engaging in natural language interactions with contact center agents, for example, or issuing and answering queries from a certified knowledge base, intelligent bots are integral for not only automating these data exchanges, but also implementing the ensuing action required to complete workflows.
“Over the next one to two years we’ll see tens of thousands more knowledge workers deploy digital assistants to reduce complexity, achieve error-free work, help their customers by drastically reducing their ‘on-hold’ times and, most importantly, eliminate the frustration that arises from performing repetitive, manual tasks,” presaged Automation Anywhere CTO Prince Kohli.
These capabilities, of course, are naturally augmented by coupling intelligent bots with the sundry of Artificial Intelligence manifestations that are more pervasive today than they ever were before. What will likely change in 2022, however, is the variety of AI that’s invoked, which is subtly shifting from pure connectionist approaches involving machine learning to a return to AI’s classical roots in symbolic reasoning.
“The real story here is sure, there have been some amazing advances in machine learning with respect to Natural Language Processing, and the results of things like GPT-3 are super impressive,” acknowledged Franz Knowledge Engineer Richard Wallace. “But there’s still a need for the old fashioned symbolic AI Natural Language Processing using rules and hand-crafting those rules, especially when you need to provide contractual or legal guarantees about what the results will be.”
Conversational Bots
With the proliferation of digital assistants such as Siri or Alexa in personal and professional realms of life, many knowledge workers consider chatbots a mere template-based, passé version of what bots are capable of achieving. However, the use cases for these linguistically savvy bots are burgeoning to include internal and external applications. According to Kohli, “Today, many knowledge workers face increased complexity due to the number of applications and devices they must access every day to perform their work. An example, though not the only one, is customer service agents or contact center employees.”
Additionally, the redoubled worth of contemporary chatbots is also attributed to the mammoth expansion of the templates they’re based on which, when involving linguistic reduction rules, is limitless. Reduction rules are a canny way of expressing the most convoluted sentence in a basic form that bots can understand—without exhaustive taxonomies. “When people are talking casually they tend to say things using a lot more words than are really necessary to convey the absolute, simplest logical statement they’re trying to make,” Wallace explained. “Reductions are a way of using patterns to recognize more complex ways of expressing things and simplifying them into simpler, logically equivalent statements.”
Symbolic AI
As Wallace’s quotation suggests, linguistic reductions are rooted in symbolic reasoning and a codified set of rules for taking certain inputs, such as “I just”, and transferring them to defined outputs which, in this case, would simply be “I”. According to Wallace these rules are recursive, enabling a series of reductions to be applied to any sentence to extract its basic meaning. This utility immensely benefits chatbots because it “applies to almost all spoken language and to Natural Language Processing in general,” noted Franz CEO Jans Aasman. Moreover, because reduction rules are orthogonal to taxonomies, they’re horizontally applicable and as useful for healthcare use cases as they are for insurance ones or any business domain’s.
It’s important to realize reductions work with—and provide greater merit when paired with—taxonomies; they operate on text as well as speech (the latter of which may involve transcribing speech to text). Additionally, use cases for equipping bots to parse natural language via this type of symbolic reasoning include pivotal regulatory compliance, data privacy, and legal areas like reading through contracts, for example. “It’s the same type of Natural Language Processing where you are looking at a paragraph of text and you can apply reduction rules to each individual sentence in the text to reduce it to its simplest form,” Wallace commented. “Then, you extract semantic relationships and represent the paragraph as a selection of triples in the database.”
Once bots understand the natural language they parse, they can respond to customers’ needs with defined replies or highlight parts of a passage relating to any number of regulations and legal demands. Best of all, they can take action to fulfill requests like initiating refund processes for returns, for example. In such cases, “Software bots perform the heavy lifting behind the scenes, creating an intuitive single-pane-of-glass, and reach into other applications to fetch data to display or to update databases, triggering other processes and following them to completion,” Kohli mentioned.
Dynamic Question Answering
Fortifying the natural language interactions of intelligent bots with an enterprise knowledge base maximizes their ability to serve knowledge workers. Wallace implied bots can insert their findings from reading text via linguistic reductions into a knowledge graph to expand the amount of enterprise knowledge about a particular subject, such as documents that may be applicable to CCPA regulations. Furthermore, by storing that knowledge as simplified subject, verb, object statements (triples) in this graph framework, it can be queried and accessed for any purpose. Holistic taxonomies according to domains or subject matter are instrumental for increasing the complexity of the answers bots can derive from this information.
This way, it’s possible to “link up chatbots and reduction techniques to questions you might ask a knowledge graph,” Aasman revealed. “For example, you ask the Wikipedia or the DBpedia ‘what is the capital of x?’. The pattern would just be ‘what is the capital of X’ and [the bot] would recognize if someone asks this thing, you have to do this kind of query in the knowledge base and find country X, and for country X find the capital.” This same approach is helpful for looking up privacy and compliance regulations about specific clauses or phrases found in documents, as well as information customers might ask contact center agents about pertaining to various products or services.
Bettering, Not Replacing Humans
Despite their efficacy for implementing swift action in these use cases and a host of others, it’s of paramount importance to realize that intelligent bots are a vital supplement, not replacement, for knowledge workers. “Ever since we started working on chatbots and then started talking about what a chatbot is with people, especially business people, a lot of times the very first thing that comes to mind is, ‘this would be great for a call center’,” Wallace recollected. “‘We can replace all the employees in our call center with these chatbots and save ourselves a ton of money.’”
Rarely, however, is such a plan actuated successful—or even completed. Though perhaps underrated, human involvement is almost always needed in most deployments. “The human touch is extremely important, even more important than the machine stuff,” Kohli posited. “Machines are there to help the agents do a better job of what they’re doing.” In some cases that may be to assess the compliance risk of a particular document or processes involving PII. In others, it may be to discuss an assortment of options with a customer for upgrading their services.
Either way, manually retrieving data with bots is merely part of what’s required for knowledge workers to do their jobs well. With certain contact center conversations “you want the agent to spend time with you and provide understanding, not have to look up data all the time,” Kohli denoted. “They should be able to give you a nice, good answer, take a look at your history, [and] perform analysis.” Employing intelligent bots to facilitate the background information necessary for agents to do so is crucial to optimizing knowledge workers’ abilities to perform their jobs well in these circumstances.
The Next Level
There’s little doubt that by implementing linguistic reduction rules, symbolic reasoning, and other NLP techniques, intelligent bots can help knowledge workers of all types do their work faster, more thoroughly, and more effectually than they can without them. Moreover, there’s little doubt that advancements in neuro-symbolic AI can expedite the population of the knowledge base required to devise rules for reductions, bots’ responses, and other dimensions of symbolic reasoning.
The next level for intelligent bots, however, is understanding entire stories customers might have and eliciting appropriate responses, which is where most fail—and where the linguistic reductions of symbolic AI has the most to gain. “That’s the leading edge of research on chatbots,” Wallace indicated. “Getting them to model little stories that people tell: little paragraphs that are multi-sentence inputs that you have to combine the meaning across the sentences to make it come up with a reasonable response.”
About the Author
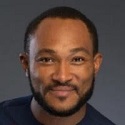
Jelani Harper is an editorial consultant servicing the information technology market. He specializes in data-driven applications focused on semantic technologies, data governance and analytics.
Sign up for the free insideBIGDATA newsletter.
Join us on Twitter: @InsideBigData1 – https://twitter.com/InsideBigData1
Speak Your Mind