Predictive analytics’ application is unlimited, from helping determine inventory needs in retail to predicting patient needs in hospitals. One ever-growing issue predictive analytics is helping to solve is the growing college drop-out rate, which is about 33% of students.
For higher education institutions, the pressure is increasing to meet enrollment numbers, retain current students and ensure those students make it to graduation while also working under tight budgets. Successfully navigating the mounting list of challenges is no easy task, but predictive analytics can ease some of this burden.
The higher education industry has coined the term ‘summer melt’ to describe enrolled students leaving for the summer and failing to return for classes in the fall. In fact, colleges report losing 10-20% of their students to this phenomenon. However, by using data analytics to gain deep student insights, colleges have been able to make smarter enrollment choices and decrease the threat of summer melt.
Predictive analytics is an untapped resource for many colleges and universities faced with the task of improving college retention. Through the compilation of data, analytics and automation, institutions gain insights that can lead to well-informed enrollment decisions and the ability to analyze the trajectory of each student’s success in advance and intervene before it is too late. This technology can lead to increased conversion rates and better-informed proactive outreach — core components needed to effectively address the growing drop-out rate. Now, let’s discuss why and how predictive analytics can better student retention efforts:
Increased Conversation Rates
For higher education institutions, predictive analytics can assist in meeting enrollment goals. For instance, colleges and universities that aim to increase conversion rates in the enrollment process can leverage big data and predictive analytics to ensure that those enrolled have a high chance of staying at the institution and completing their higher education journey at a school.
You’re probably wondering how and what insights are compiled to form a prediction regarding a student’s success. To answer this question, the technology analyzes a combination of data points that include metrics such as academic interests, engagement, test scores and GPA. The compilation of these individual data sets are then leveraged through Enterprise Data Systems where predictive analytics steps in to make automatic, data-driven determinations on which individuals present the highest predictive strength.
With admissions recruiters reviewing thousands of applications, predictive analytics and automation can assist in determining the students that have the highest likelihoods of retention. As colleges and universities navigate budget restrictions, limited staff and a growing list of additional responsibilities, every enrollment decision needs to be strategic, intentional and well-informed if they want to maximize success as an institution. Predictive analytics makes that possible.
Better-Informed Proactive Outreach
Predictive analytics isn’t only useful for enrolling new students, but also retaining current ones. Having a predictive solution that notifies professionals and the individual students of their change in performance can allow institutions to intervene before it is too late. The importance of early intervention and closed-loop transparency is crucial, as research shows that students who drop out are likely to never return.
For community colleges in particular, students’ personal lives vary dramatically – some may have children, families, full-time jobs, you name it – which place them at a higher risk of falling behind and placing other life aspects in front of their education. With predictive analytics, performance indicators and student data are pulled from enterprise resource planning (ERP) systems, learning management systems (LMS) and additional outlets that house information that can inform predictions regarding risk of attrition, like student interactions with staff.
Having a data-driven solution gives college staff and students the right information at the right time. With this, the solution can alert the student of their performance and hold them accountable at every step. Another aspect of predictive analytics for outreach is the concept of risk factors. Big data is utilized to place students in risk categories: Green (Low Risk), Yellow (Medium Risk) and Red (High Risk). When a student experiences a change in circumstance or performance, that risk level may change. Through automation and big data analysis, staff members can instantly be notified of the change and initiate proactive outreach to assist in resolving the issue causing a negative impact to their performance and success.
Predictive analytics gives college and university staff the ability to read between the lines of each student, helping to enroll students that show promise of completion and targeting when a student needs help before it’s too late. As we navigate an evolving landscape and higher education environment, leveraging predictive analytics can help not only provide students with crucial support, but set institutions up for long-term success.
About the Author
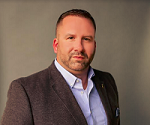
Bryan Bell is the Chief Data Scientist at Watermark Insights, where he is responsible for analyzing data and ensuring that Aviso Retention’s efforts are adequately aligned with the needs of students and education leaders. A solutions-driven data scientist and entrepreneur with a passion for data storytelling, Bryan uses data science to support the overall mission of Aviso Retention. He is also responsible for the Aviso Predict product, a collection of risk models created using data science methods to describe risk at Institutions of Higher Education.
Sign up for the free insideBIGDATA newsletter.
Join us on Twitter: @InsideBigData1 – https://twitter.com/InsideBigData1
Gartner rightly predicted that business users in every industry and vertical enterprise would become Citizen Data Scientists, using data to help refine target audiences, and make the right decisions. Analytics that can be easily integrated with best-of-breed industry software and used by business users who have domain knowledge but are NOT data scientists will drive this shift. Every organization that wants to embrace this new reality will need to make a cultural shift in order to encourage business professionals to embrace and use data to make fact-based decisions. Courses that allow users to see augmented analytics in use and practice and to understand basic concepts and their new role will help businesses and industries to make this change.