Traditionally, data strategy has involved striking the right balance between diametrical forces, needs, and enterprise desires. Examples of this dichotomy include the time-honored notion of offense versus defense, in which organizations balance opportunities for monetizing data with managing data’s risk. Others include positioning and processing resources in the cloud versus doing so on-premises, or counterbalancing the use of manual methods with automated ones.
This sense of duality characterizing data strategy will endure throughout 2023, and likely well beyond. What’s new, however, is the aspects of data management to which it’s applied. The preeminence of cloud processing and storage is readily apparent. However, it’s left vital questions to be answered, such as how much (and which) data should be managed locally, in distributed settings, as opposed to doing so in centralized ones.
Tantamount to this strategic concern is that for determining which data-driven actions should be predicated on real-time (or predictive) analysis versus those based on historic analysis. And, most prominently, there’s the issue of data ownership on the sides of organizations and consumers, which is perhaps the nexus point for data privacy, regulatory compliance, and consumer rights.
The answers to these questions involve constructs like data lake house, data mesh, and data fabric architectures, pragmatic concerns like a business semantics layer, established applications like digital twins and the Internet of Things, and novel approaches around the metaverse and data clean rooms.
Moreover, there is an increasingly strategic need to personalize interactions and even downsize data amounts.
“If you saw the Databricks versus Snowflake kind of benchmark wars, the data size that they chose for that was 100 terabytes,” recalled Jordan Tigani, Motherduck CEO. “That’s a data size that virtually nobody has, nobody uses, and nobody necessarily cares about. The size of data that customers actually have is many orders of magnitude smaller.”
Distribution vs. Centralization
As Tigani alluded to, oftentimes the data required for a specific workload related to individual domains like sales or marketing isn’t necessarily sizable. Nevertheless, such data are often vastly distributed throughout multiple cloud or on-premise localities. Storing, processing, and querying such data effectively centers on a principal data strategy consideration about where to do so.
Although massive centralized warehouses like BigQuery won’t become obsolete anytime soon, there are mounting reasons for accessing and processing data locally. “When you spin up a normal virtual machine on AWS, it’s got 64 cores and 256 gigs of RAM,” Tigani indicated. “That’s massive. Very few workloads can’t fit in that. That’s roughly equivalent to a Snowflake XL that’s half a million dollars a year.” Other compelling reasons for processing data locally in distributed settings include:
- Edge Computing: Cogent cloud solutions enable users to store and query data on-demand on their existing hardware (like laptops), while scaling up and supporting user concurrency with instances the precise size of their workloads. “If you accept that data sizes are going to be smaller, you push the data out to the end user,” Tigani observed. “Laptops used to be underpowered. Now, they’re supercomputers.”
- Minimal Data Movement: Query federation tools that minimize data movement—and defer movement until the last possible moment—also reduce data pipeline costs while reinforcing data governance and regulatory compliance. Consequently, mechanisms like Trino, Presto, and others are gaining credence as part of the data mesh and data fabric architectures.
- Low Latency: The negligible latency of edge processing has always been one of its advantages, especially with on-demand, query-in-place options in the cloud. Such offerings enable “someone with a smaller set of data to be able to manage that data and collaborate on it and query it very fast,” Tigani maintained.
Comprehensive Semantic Layer
Implementing a business semantics layer has become practically ubiquitous throughout data management, regardless of vertical or vendor type. Almost everyone, from Business Intelligence to data virtualization sellers, is utilizing such a layer to standardize data’s meaning according to business-friendly terms. This layer is pertinent for attaching rigorous data governance to a data lake house, data lake, and data fabric while democratizing data’s use. “Once you have that, you can use that metadata,” Franz CEO Jans Aasman denoted. “BI can use that metadata. Reports can use the metadata. Data science and machine learning can all use the same metadata layer when getting data.”
The fundamental components of a semantic layer consist of an ontology or subject area model describing the business concepts (and words for them) to which data pertains. There’s also a cataloging element that describes where, and what, all those data are. Lastly, “there’s the linkage between business concepts and the [Digital Assets Management] catalog where…I find the information about the concept in my database,” Aasman added. When combined with query-in place capabilities, semantic layers foster environments in which “emerging software architectures in 2023 will be built around data quality, data pipelines, and traceability, rather than computing, processing, APIs, and responses,” mentioned Ratnesh Singh Parihar, Talentica Software Principal Architect.
Real-Time vs. Historic Analysis
The influx of real-time data from sources such as the Internet of Behaviors and Industrial Internet is shifting analytics from historic to low latent applications. Granted, synthesizing these types of analytics can only improve the capacity to attain universal goals such as supply chain management, impact analysis, product development, and customer satisfaction. However, real-time analytics’ worth is expanding in this regard, particularly in relation to digital twins “which we can put atop a real world system with real data coming through…[to] tell us if a potential problem could happen,” explained Dan Mitchell, SAS Global Director of Retail and CPG Practice.
Conventionally, this functionality was reserved for predictive maintenance use cases in manufacturing lines or industrial equipment assets, like trains. In 2023, use cases will broaden to include dynamic models of enterprise data systems for data governance. Digital twins of supply chain networks are primed for instant responses to market conditions, as well as impact analysis and testing to predetermine responses. Mitchell referenced 2022’s baby formula crisis as a good example of a situation in which digital twins could have “run scenario analysis to predict how to best solve… that problem,” before hundreds of thousands of parents were affected.
Data Ownership, Data Privacy, and The Metaverse
The enterprise merit of the digital twin proposition increases exponentially when placed within the metaverse, which Mitchell defined as “the Internet of Place and People. With the metaverse there will be places that you go to and the experience, data, and value you receive will be about [that] place.” Virtual Reality, Augmented Reality, and smart phone devices will let users access the metaverse. Placing digital twins inside the metaverse has significant consequences, particularly for product or service development, testing these developments, customer interactions, data privacy, and data ownership. This tandem has immediate implications for:
- Employees: Organizations can build digital twins of physical facilities to train employees on new equipment. Maintenance personnel can interact with digital twins of assets like vehicles to perfect repairs or implement predictive maintenance. Generative AI can help by creating different product or feature variations for market research groups. According to Talentica Principal Data Scientist Abhishek Gupta, “High resolution images generated from a few lines of text has the potential to transform visual creative industries like advertising, as well as spur new ideas for products.”
- Customers: In the metaverse, organizations can “transact with people directly without anybody in the middle,” Mitchell remarked. Conventional ‘middlemen’ and payment methods (like credit cards) will be replaced via Non Fungible Tokens, Fungible Tokens, and cryptocurrencies.
- Data Ownership: The metaverse supports a paradigm in which “we will see Blockchain data sharing system architectures empower users to gain control over the ownership of their data,” said Talentica CTO Manjusha Madabushi. “Users will…decide who has access to it.” The indisputable nature of blockchain solidifies consumer data ownership so data subjects can “apply that to their identity, personal data, and privacy,” Mitchell indicated.
- Data Clean Rooms: Although data clean rooms aren’t expressly related to the metaverse, they’re a credible means of inter-organizational analytics while enforcing data privacy. With this invention, companies “set up a system, a cloud app, database, whatever you want to call it, where through security I can put my data in, you can put your data in, we’re not sharing the data with each other, and we can both query it,” Mitchell revealed.
Over Time
A holistic data strategy is inseparable from prudent data management, especially for fulfilling enterprise objectives in a sustainable manner over time. Although that strategy will differ according to each organization, collectively, such strategic measures may rely less on replicating data for centralized approaches, and more on querying data in place via distributed methods.
The reusability of a semantic layer will solidify the necessities of aggregating or integrating such data. The transformational nature of the capacity of digital twins to provide real-world simulations in digital settings—such as the metaverse—must be incorporated into organizations’ strategic concerns.
If not, it may be for their competitors’, at the costly expense of competitive advantage.
About the Author
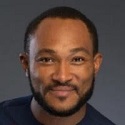
Jelani Harper is an editorial consultant servicing the information technology market. He specializes in data-driven applications focused on semantic technologies, data governance and analytics.
Sign up for the free insideBIGDATA newsletter.
Join us on Twitter: https://twitter.com/InsideBigData1
Join us on LinkedIn: https://www.linkedin.com/company/insidebigdata/
Join us on Facebook: https://www.facebook.com/insideBIGDATANOW
Speak Your Mind