Healthcare’s adoption of artificial intelligence (AI) and machine learning (ML) has accelerated quickly. Data analytics has transformed the industry with insights from the information it gathers influencing how medical researchers, physicians, policy advocates and patients operate within the system. Data gleaned from AI improves healthcare in many areas, including:
- How organizations conduct medical studies.
- Patients’ understanding of the costs of health insurance and medical tests.
- Physicians’ abilities to make preventative recommendations to patients.
Organizations also leverage this data to understand patient pain points, identify where call center agents need more training, and uncover insights from customer experience and marketing.
But as the scope of data increases (with over 200 zettabytes of data in cloud storage worldwide by 2025), healthcare organizations will need more efficient methods to collect, evaluate and analyze it to gain insights for informing strategic decision-making.
AI’s contribution to data analysis
AI-driven tools play an increasingly significant role in analytics, including those used to improve customer experience, by extracting valuable, actionable data insights. AI and ML enable healthcare organizations to more effectively listen to — and better understand — their customers’ voices and solve common issues or barriers, like the “Eddy Effect,” disrupting customer interactions.
There is, however, a caveat: An ML model’s reliability is only as good as the dataset on which it’s trained. These training and learning datasets are essential to ML models, helping them make accurate predictions and perform desired tasks. ML relies on comprehensive, relevant, representative and uniform training data generated from unstructured data. The best ML models have a 70% to 90% accuracy rate.
In healthcare, this unstructured data comes from sources, such as healthcare-focused conversations. The right technology can collect, evaluate and analyze conversational data at scale.
Conversational data generated via call centers, chatbots and emails — and analyzed via AI, ML and natural language processing (NLP) — will become increasingly critical in helping organizations assess, understand and resolve CS issues. Unlike traditional NPS and surveys, conversational data provides more nuanced, contextual and richer insight across diverse business functions. It enables organizations to:
- Identify customer pain points and challenges.
- Predict future events.
- Automate work processes and streamline systems.
- Enhance personalization.
The value of qualitative and quantitative insights
Data-backed storytelling combines quantitative and qualitative data to provide meaning. When it’s brought to life, data enables people to connect to concepts that they find most valuable and personal. Quantitative data helps create concrete information in numerical form, while qualitative data helps create understanding and context behind the numbers.
Imagine this scenario: a life sciences company sought solutions for improving its patients’ journeys and standardizing call center agent messaging. While they’d previously gathered insights from a small sampling of patient calls, the data wasn’t fully representative.
The company adopted AI technology to analyze all conversational data from when patients began therapy to their last communication, explicitly focusing on patient journeys with high call volumes. The analysis enabled the organization to identify where:
- Agent messaging lacked consistency, causing patient stress or confusion.
- Patients developed routine or confusion (The Eddy Effect) within the journey.
- Opportunities existed for the organization to provide additional support to caregivers and patients.
To identify the challenges these patients faced, the organization applied qualitative and quantitative approaches to the data it collected and analyzed. While these approaches appear to be opposite sides of the same coin, they complement each other, blending head and heart, big data and data immersion, and AI and humans, enabling organizations to tell a contextual, data-backed story. Storytelling is integral to understanding insights. Why? Because storytellers (those who analyze the data) can analyze all the touchpoints in a patient’s journey to understand each patient’s barriers.
Listening at scale helps solve common issues
While contact center agents generally focus on helping patients solve problems one interaction at a time, conversational intelligence enables organizations to hone in on systemic issues. AI identifies data and insights on the macro and micro levels, from issues preventing patients from getting what they need to interactions on a thematic level.
Listening directly to patients’ voices enables healthcare leaders to maximize the data their organizations have already collected to deliver insights and ROI. Comprehensive, actionable patient feedback derived from conversational data empowers leaders to create and implement patient-centric strategies. This strategy goes beyond meeting customer expectations — and provide the most effective support. The best way to achieve this goal? By listening at scale.
About the Author
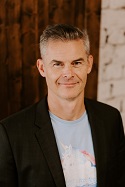
Michael Armstrong is the Chief Technology Officer at Authenticx and a foundational leader in building our solution infrastructure. In this role, he leads a team of data engineers and scientists to translate big visionary ideas into practical and actionable software. Michael has extensive experience in engineering, data architecture, product development, and business intelligence.
Sign up for the free insideBIGDATA newsletter.
Join us on Twitter: https://twitter.com/InsideBigData1
Join us on LinkedIn: https://www.linkedin.com/company/insidebigdata/
Join us on Facebook: https://www.facebook.com/insideBIGDATANOW
Speak Your Mind