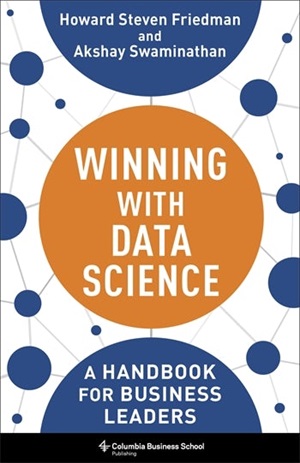
Many businesses fail to see value from their investments in data, analytics and AI resulting in wasted money, time and opportunity for the company.
The main reasons data science efforts fail can be categorized as business-related or data-related. In this second of two articles, we will describe some of the most common data-related reasons for these failures and what you can do to avoid these pitfalls.
Data science teams are not just project performers, waiting to receive instructions. They should be listeners, problem solvers and guides. They are the business customers’ collaborative partners. Good data scientists listen to customer’s needs, invest time, ask the right questions, patiently answer technical questions, challenge assumptions, and speak in a language that non-specialist can understand.
Some important data-related mistakes in data projects include:
- Ignoring the project aspects: Data science projects are just that, projects. The same basic principles of defining success, communicating regularly with clients and developing strong documentation are critical. Agreeing on the final product, expected usage, timelines and costs should happen at the outset and you should always remember that the documentation needs to be sufficiently transparent so that someone else can readily step into the project and understand the data sources, code, feature engineering and modeling details.
- Starting with the solution instead of the problem: The goal of any data science project should be to solve a pressing business problem. Often, data teams make the mistake of starting with the solution—a new model architecture, a new data source, a new dashboard tool—without understanding whether or not there is a business need or end user asking for it. This can lead to wasted effort, resources, and time. Don’t take a hammer and go looking for nails. Instead, partner with business stakeholders to identify a real problem and pick the appropriate tool to solve it.
- Skipping the data quality checks: “Garbage in garbage out” is a mantra in data science, yet it is often forgotten. Some of the most common mistakes include failing to include data quality checks at the beginning as well as not identifying and appropriately handling missing data. More generally, using dirty data with errors, inconsistencies, and missing values leads to unreliable or biased models and flawed insights.
- Not visualizing data: The human eye is a powerful tool yet too often data scientists want to jump into modeling without pausing to look at the data itself. Relying solely on numerical summaries overlooks valuable patterns and relationships that can be uncovered through visualizations.
- Poor model monitoring: Drift happens. Data drift related to the features and model drift in terms of declining performance must be measured. That said, there are still data science teams that launch models then forget. The business then becomes the one to knock on the door and remind them that the model is no longer delivering at its previous levels. That’s a knock you don’t want to answer.
- Feature Engineering Errors: Issues with feature engineering, selecting irrelevant features, or introducing leakage can significantly hurt model performance in the short term and result in model performance deteriorating rapidly. Model interpretability can be hindered by poor feature engineering resulting in customers losing confidence in the output and data leakage can cause issues for the model performance and the modeler’s credibility.
- Overfitting models: Poor practices related to validation and testing can lead to models that are overfit. Their performance on training data ends up being vastly superior to that of naive data sets. Practically speaking, this means that when the models are introduced into the real world, the performance is far worse than expected leading to customer disappointment.
- Neglecting security: No one wants to be the lead story on the news due to a data breach. Spyware, malware and other hacking attacks occur constantly, and the data science team needs to be vigilant. Failing to secure data access and storage can lead to sensitive information being compromised along with the reputation of the company and the data scientist.
All of these mistakes stem from failures in “dotting the i’s and cross the t’s”. A well-trained data scientist is aware of these important steps, yet they are skipped sometimes due to time pressure or sometimes simply a failure to follow best practices. This is why our first item on the list is about project planning. Before initiating a data science project, we recommend the data scientist takes the time to meet with the business customer to understand the details of the project goals, constraints and how success will be measured. Following that step, the best practices in data science can be folded into the timelines.
Adapted from Winning with Data Science by Howard Steven Friedman and Akshay Swaminathan, published by Columbia Business School Publishing. Copyright (c) 2024 Howard Steven Friedman and Akshay Swaminathan. Used by arrangement with the Publisher. All rights reserved.
About the Authors
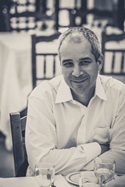
Howard Steven Friedman is a data scientist, health economist, and writer with decades of experience leading data modeling teams in the private sector, public sector, and academia. He is an adjunct professor, teaching data science, statistics, and program evaluation, at Columbia University, and has authored/co-authored over 100 scientific articles and book chapters in areas of applied statistics, health economics and politics. His previous books include Ultimate Price and Measure of a Nation, which Jared Diamond called the best book of 2012.
.
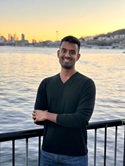
Akshay Swaminathan is a data scientist who works on strengthening health systems. He has more than forty peer-reviewed publications, and his work has been featured in the New York Times and STAT. Previously at Flatiron Health, he currently leads the data science team at Cerebral and is a Knight-Hennessy scholar at Stanford University School of Medicine.
.
Sign up for the free insideBIGDATA newsletter.
Join us on Twitter: https://twitter.com/InsideBigData1
Join us on LinkedIn: https://www.linkedin.com/company/insidebigdata/
Join us on Facebook: https://www.facebook.com/insideBIGDATANOW
Speak Your Mind