Not having an artificial intelligence solution to combat bad data in 2021 is like missing the internet trend in the 1990s. It’s no longer a question of whether a business wants to go this route, the use of machine learning has become a necessity born out of the amounts of data organizations collect annually which makes it impossible for data scientists to navigate manually.
Inefficient data cleaning processes cost sales and marketing departments around 550 hours of wasted manpower and as much as $32,000 per sales rep a year. More alarmingly, it costs businesses $3 trillion a year in lost revenue. As companies scale in size at a record breaking pace, growing their databases by tens of thousands of new users year over year, a whopping 30% of data becomes inaccurate every year. The need for efficient, effective, and scalable data cleaning solutions is therefore one expense companies can’t afford to cut back on.
Yet despite these figures and AI’s promise of combating the perils of bad data, a 2019 Forrester report on data quality indicates businesses across the SaaS spectrum are still falling short of utilizing AI optimally. With 75% of SaaS executives believing the fate of their companies rests on the quality of their database, what is it that’s stopping companies from using AI technologies in an optimal fashion that really brings down the costs of bad data?
Siloed Workflows Decrease the Effectiveness of AI Solutions
The answer is grounded, primarily, in the workflows that accompany AI-powered solutions.
Deriving value from AI and machine learning technologies crucially depends on the workflows that accompany its adoption. Organizations that take on AI must first rewire their interdepartmental collaborations at the workflow level and break through existing organizational barriers, most crucially siloed work environments.
Artificial intelligence affects ‘small unit’ problems such as database segmentation, as well as ‘big picture’ problems such as optimizing an entire customer journey. To get the full effect of deep learning and advanced analytics, organizations must strive to see the big picture at all times while forcing all departments to look at the granular level as well.
AI Comes with Reframing Mistakes as Learning Opportunities
This idea follows from the previous one relating to the time needed for AI results to fully take shape. Because AI rests on recognizing data patterns and the development of rules that apply from individual case to database wide, organizations must be agile and experimental enough to apply AI findings from one section of their operation across their entire business. Risk-averse, rigid organizations stifle AI’s learning capabilities and prevent these findings from improving results across the board.
Deep learning adoption requires that organizations allow certain bandwidth for test-and-learn processes. We must remember that on the first iteration, AI applications rarely have all their desired functionality. Listening in on early user experiences and being attuned to a continuous feedbacking cycle will allow AI systems to refine their capabilities over time and deliver more accurate results.
AI is Not a Plug-and-Play Technology
Companies expect immediate results and when they don’t see them they fall short of applying AI to broader parts of the organization. Cleaning up databases takes time because it requires learning the patterns of your data before applying cleaning processes.
It is impossible to know the data quality problems hidden in your database prior to the adoption of AI solutions. A set of infrastructure rules and quality constraints must first be determined before these can be applied to the whole business.
Time and gradual building is the name of the game when adopting new technologies, and it’s most certainly the case with enjoying the full range of AI’s functionability.
Final Thought
In an automation-driven business world, humans must work alongside machines to make profit happen. Artificial intelligence is just one example of the way machines are at the service of all parts of an organization’s overall utilization.
Primarily, they force businesses to break down departmental silos and promote interdepartmental collaborations that yield better ideas and solutions. Secondly, they empower workers on all levels to make independent decisions backed up by data. Finally, they teach organizations and the people working in them about flexibility of mind, about adaptive behaviors and about making changes on-the-go as AI insights come in continuously, shaping and reshaping business plans.
About the Author
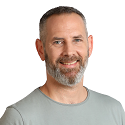
As VP of R&D, Shai Gottesdiener serves as a strategic technology executive, leading the technical delivery of Lusha’s product, IT, and information-security. With over 20 years of experience in the internet and enterprise software industry, Shai also served as CTO of both Perion and Selina and VP of R&D at 888, before joining Lusha. Shai holds a B.A. in computer science and logistics from Bar Ilan University.
Join us on Twitter: @InsideBigData1 – https://twitter.com/InsideBigData1
Sign up for the free insideBIGDATA newsletter.
Speak Your Mind