AI has become the norm for enterprises across every industry. According to a recent report by Gartner, 75% of businesses are expected to shift from piloting to operationalizing AI by 2024. It’s not surprising to see more companies turning to the technology; AI has the potential to provide innovative solutions to our everyday problems, and we’ve even seen cases of AI techniques detecting the early onset of diseases like COVID-19.
For each high-profile case that comes under public scrutiny, the problem remains the same: a lack of model transparency. Many of the AI models businesses deploy today are considered to be opaque, which often leads to issues being detected after decisions have been made and people have already been affected. Model Performance Management (MPM) helps organizations operationalize AI in a safe and trustworthy way. It goes beyond metrics to also explain results. Think of responsible AI as the core underlying layer, as the fundamental layer of MPM.
Responsible AI is the practice of building AI that is transparent, accountable, ethical, and reliable. When AI is developed responsibly, stakeholders have insight into how decisions are made by the AI system, and the system is governable and auditable through human oversight. As a result, outcomes are fair to end users, stakeholders have visibility into the AI post-deployment, and the AI system continuously performs as expected in production.
Implementing responsible AI not only saves enterprises from being scrutinized for biased machine learning algorithms, but also enables enterprises to make better, truly informed decisions. As AI in the enterprise continues to grow, it is imperative that organizations ensure they’re following responsible, ethical, and transparent AI practices at all times.
Here are three ways responsible AI can benefit the modern-day enterprise.
1. Reduces instances of AI bias
There are calls for increased transparency from within the AI industry, but the challenge is that AI systems are prone to bias. These risks most often show themselves in the forms of headline-making instances, such as Apple’s application process for the apple card and Zillow’s AI-enabled iBuying program. In the case of Zillow, the company failed to predict house price appreciation accurately. The company intended to use ML models to buy up thousands of houses per month, whereupon the homes would be renovated and sold for a profit. Unfortunately, things didn’t go to plan. News came out that the company was shutting down its iBuying program as it overpaid thousands of houses due to its pricing algorithms. Both Apple and Zillow are unfortunately examples of companies who, had they implemented responsible AI frameworks, could have been shielded from business, operational, ethical, and compliance risks.
2. Drives better data driven decisions
Data drift occurs in a model over time when the data provided to a model to make a decision changes slightly or when new data emerges. This causes the model’s performance to degrade if it is not updated or if it goes unnoticed—which could ultimately lead to poor, inaccurate decisions that negatively impact bottom lines, customer experiences, and more.
Understanding why different models are predicting what they are predicting is a very important part of analyzing model behavior. Having this level of insight is becoming increasingly more important; an IBM survey found that 84% of IT professionals now say being able to explain how their AI arrives at different decisions is important to their business. This not only drives better decision making, but also prepares organizations for questions on why their models are performing the way they are as AI regulations become the norm.
3. Prepares organizations for AI regulations
Following the EU’s implementation of AI regulation in 2021 and the national security commission of AI, companies leveraging AI in everyday business activity are bound to eventually need to comply with specific regulations (if they don’t already). Most experts expect to see this wave of regulations grow over the next few years. Beyond the societal and business risks of implementing opaque AI, enterprises are at risk of losing money via fines and being non-compliant. Taking too long to validate or not being able to generate explanations are costly for businesses, and in some instances, can lead to revenue losses.
To prepare for that inevitability, it is essential that every company consider how responsible AI can ensure that their algorithms are free of biases. Focusing on bias detection and explainable AI can help organizations prepare to demonstrate the fairness and accuracy of their algorithms in preparation for any future regulations that will be put in place.
Start building a culture for responsible AI
AI impacts lives, making it imperative that the system is governable and auditable through human oversight. In order to successfully leverage the power of AI to grow businesses while ensuring that AI outcomes and predictions are accurate, ethical, fair, and inclusive, modern enterprises will need to have a greater focus on building AI that is transparent. With a responsible, transparent AI solution in place that can actively monitor and manage models in production, executives can feel confident in their organization’s ability to eliminate bias, data drift, and risks of non-compliance—while capitalizing on the benefits of AI in driving business forward.
About the Author
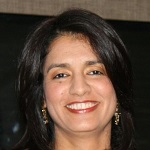
Kirti Dewan, VP of Marketing of Fiddler AI. Kirti has over 20 years of experience in the technology sector and has worked in marketing leadership and product marketing roles at Bugsnag, EngineYard, and VMware to name a few. She is excited to help companies deliver better AI outcomes for society at large.
Sign up for the free insideBIGDATA newsletter.
Join us on Twitter: @InsideBigData1 – https://twitter.com/InsideBigData1
Speak Your Mind